"Large-Scale Deep Learning for Building Intelligent Computer Systems," a Keynote Presentation from Google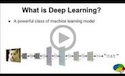
Jeff Dean, Senior Fellow at Google, presents the keynote talk, "Large-Scale Deep Learning for Building Intelligent Computer Systems," at the May 2016 Embedded Vision Summit. Over the past few years, Google has built two generations of large-scale computer systems for training neural networks, and then applied these systems to a wide variety of research problems. Google has released its second generation system, TensorFlow, as an open source project, and is now collaborating with a growing community on improving and extending its functionality. Using TensorFlow, Google's research group has made significant improvements in the state-of-the-art in many areas, and dozens of different groups at Google use it to train state-of-the-art models for speech recognition, image recognition, various visual detection tasks, language modeling, language translation, and many other tasks. In this talk, Jeff highlights some of ways that Google trains large models quickly on large datasets, and discusses different approaches for deploying machine learning models in environments ranging from large datacenters to mobile devices. He then discusses ways in which Google has applied this work to a variety of problems in Google's products.
Dyson Demonstration of its 360 Eye Robot Vacuum Cleaner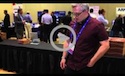
Mike Aldred, Electronics Lead at Dyson, demonstrates the company's 360 Eye robot vacuum cleaner at the May 2015 Embedded Vision Summit. The 360 Eye uses computer vision as its primary localization technology. A decade in the making, it was taken from bleeding edge academic research to a robust, reliable and manufacturable solution by Aldred and his team at Dyson.
More Videos
|
Video Stabilization Using Computer Vision: Tips and Insights From CEVA’s Experts: Part 1 and Part 2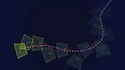
Demand is on the rise for video cameras on moving platforms, notes CEVA's Ben Weiss in this two-part article series. Smartphones, wearable devices, cars, and drones are all increasingly employing video cameras with higher resolution and higher frames rates. In all of these cases, the captured video tends to suffer from shaky global motion and rolling shutter distortion, making stabilization a necessity. Integrating an embedded video stabilization solution into the imaging pipeline of a product brings significant value to the customer. It improves the overall video quality and, at the same time, enables better video compression and more robust object recognition for higher-level computer vision tasks. More
Eye Heart VR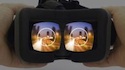
ARM’s Freddi Jeffries, in her recent blog post, looks at eye tracking and the impact it could have on the way we use VR (virtual reality) in the future. Eye tracking is not new – people have been doing it for nearly twenty years – but head-mounted displays for VR could be the catalyst that the technology needs to unlock its true potential. More
More Articles
|
Deep Learning on Embedded Systems: A Free Webinar from CEVA: July 27, 2016
A Brief Introduction to Deep Learning for Vision and the Caffe Framework: A Free Webinar from the Alliance: August 24, 2016
Deep Learning for Vision Using CNNs and Caffe: A Hands-on Tutorial: September 22, 2016, Cambridge, Massachusetts
IEEE International Conference on Image Processing (ICIP): September 25-28, 2016, Phoenix, Arizona
SoftKinetic DepthSense Workshop: September 26-27, 2016, San Jose, California
Embedded Vision Summit: May 1-3, 2017, Santa Clara, California
More Events
|