Dear Colleague,
The Edge AI and Vision Alliance is now accepting applications for the 2020 Vision Product of the Year Awards competition; the deadline is this Friday, March 20. The Vision Product of the Year Awards are open to Member companies of the Alliance and celebrate the innovation of the industry’s leading companies that are developing and enabling the next generation of computer vision products. Winning a Vision Product of the Year award recognizes your leadership in computer vision as evaluated by independent industry experts; winners will be announced at an online event on May 19, 2020. For more information, and to enter, please see the program page.
Brian Dipert
Editor-In-Chief, Edge AI and Vision Alliance |
Using High-level Synthesis to Bridge the Gap Between Deep Learning Frameworks and Custom Hardware Accelerators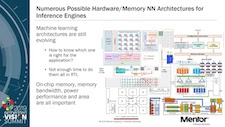
Recent years have seen an explosion in machine learning/AI algorithms with a corresponding need to use custom hardware for best performance and power efficiency. However, there is still a wide gap between algorithm creation and experimentation (using deep learning frameworks such as TensorFlow and Caffe) and custom hardware implementations in FPGAs or ASICs. In this presentation, Michael Fingeroff, HLS Technologist at Mentor, explains how high-level synthesis (HLS) using standard C++ as the design language can provide an automated path to custom hardware implementations by leveraging existing APIs available in deep learning frameworks (e.g., the TensorFlow Operator C++ API). Using these APIs can enable designers to easily plug their synthesizable C++ hardware models into deep learning frameworks to validate a given implementation. Designing using C++ and HLS not only provides the ability to quickly create AI hardware accelerators with the best power, performance and area (PPA) for a target application, but helps bridge the gap between software algorithms developed in deep learning frameworks and their corresponding hardware implementations.
Hardware-aware Deep Neural Network Design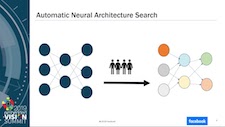
A central problem in the deployment of deep neural networks is maximizing accuracy within the compute performance constraints of embedded devices. In this talk, Peter Vajda, Research Manager at Facebook, discusses approaches to addressing this challenge based on automated network search and adaptation algorithms. These algorithms not only discover neural network models that surpass state-of-the-art accuracy, but are also able to adapt models to achieve efficient implementation on diverse processing platforms for real-world applications.
|
Selecting the Right Imager for Your Embedded Vision Application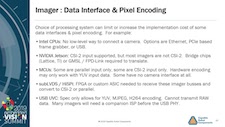
The performance of your embedded vision product is inexorably linked to the imager and lens it uses. Selecting these critical components is sometimes overwhelming due to the breadth of imager metrics to consider and their interactions with lens characteristics. In this presentation from Chris Osterwood, Founder and CEO of Capable Robot Components, you’ll learn how to analyze imagers for your application and see how some attributes compete and conflict with each other. A walk-through of selecting an imager and associated lens for a robotic surround-view application shows the real-world impact of some of these choices. Understanding the terms, the trade-off space and application impact will guide you to the right components for your design.
2D and 3D Sensing: Markets, Applications, and Technologies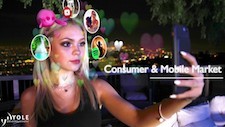
In this talk, Guillaume Girardin, Photonics, Sensing and Display Division Director at Yole Développement, details optical depth sensor market and application trends.
|