Deep Learning for Medical Imaging: COVID-19 (Novel Coronavirus) Detection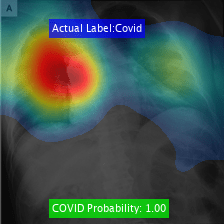
In this guest blog post published by MathWorks, Dr. Barath Narayanan from the University of Dayton Research Institute, along with his colleague Dr. Russell C. Hardie from the University of Dayton, provide a detailed description of how to implement a deep learning-based technique for detecting COVID-19 on chest radiographs. Their approach leverages MathWorks’ MATLAB along with an image dataset curated by Dr. Joseph Cohen, a postdoctoral fellow at the University of Montreal.
Vision Opportunities in Healthcare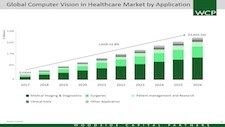
In this presentation, Vini Jolly, Executive Director, and Rudy Burger, Managing Partner, both of Woodside Capital Partners, outline trends and opportunities in computer vision for healthcare applications.
|
Addressing Corner Cases in Embedded Computer Vision Applications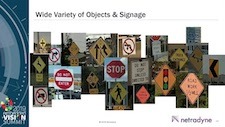
Many embedded vision applications require solutions that are robust in the face of very diverse real-world inputs. For example, in automotive applications, vision-based safety systems may encounter unusual configurations of road signs, or unfamiliar temporary barriers around construction sites. In this talk, David Julian, CTO and Founder of Netradyne, presents the approach that his company uses to address these “corner cases” in the development of Netradyne’s intelligent driver-safety monitoring system. The essence of the company’ approach is establishing a virtuous cycle which begins with running analytics at the edge and identifying scenarios of interest and corner cases on the embedded edge device. Data from these cases is then uploaded to the cloud, where it is labeled and then utilized for training new deep learning and analytics models. These new models are then deployed to the embedded device to enable improved performance and begin the cycle anew. Juian also looks at how his company uses this virtuous cycle to develop and deploy new features. Additionally, he shows how his company leverages customer expertise to help identify corner cases at scale.
AI Reliability Against Adversarial Inputs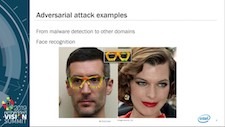
As artificial intelligence solutions are becoming ubiquitous, the security and reliability of AI algorithms is becoming an important consideration and a key differentiator for both solution providers and end users. AI solutions, especially those based on deep learning, are vulnerable to adversarial inputs, which can cause inconsistent and faulty system responses. Since adversarial inputs are intentionally designed to cause an AI solution to make mistakes, they are a form of security threat. Although security-critical functions like login based on face, voice or fingerprint are the most obvious solutions requiring robustness against adversarial threats, many other AI solutions will also benefit from robustness against adversarial inputs, as this enables improved reliability and therefore enhanced user experience and trust. In this presentation, Gokcen Cilingir, AI Software Architect, and Li Chen, Data Scientist and Research Scientist, both at Intel, explore selected adversarial machine learning techniques and principles from the point of view of enhancing the reliability of AI-based solutions.
|