LETTER FROM THE EDITOR |
Dear Colleague,
On Tuesday, September 28 at 9 am PT, Sequitur Labs will deliver the free webinar “Securing Smart Devices: Protecting AI Models at the Edge” in partnership with the Edge AI and Vision Alliance. Billions of new IoT devices are expected to come online in just the next few years. Securing these devices is an area of significant concern–to date, about half of IoT vendors have experienced at least one security breach. IoT vendors need to ensure that their products are designed, manufactured and deployed without risk of being compromised. And the problem is becoming even more serious with the deployment of AI models at the network edge. Implementing IoT security, is, however, a big challenge. It requires understanding a variety of new features and functions, a steep learning curve in learning how to implement these features using the silicon of choice, and an investment in mastering a diverse, fragmented microprocessor market, since each microprocessor vendor implements security differently. This webinar will cover best practices for securing smart devices at the edge, including a number of methods for protecting AI models, as well as real-life case studies and demonstrations of the concepts discussed. For more information and to register, please see the event page.
Brian Dipert
Editor-In-Chief, Edge AI and Vision Alliance |
NEURAL NETWORK OPTIMIZATION |
Designing Bespoke CNNs for Target Hardware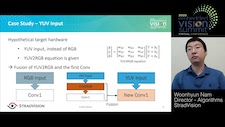
Due to the great success of deep neural networks (DNNs) in computer vision and other machine learning applications, numerous specialized processors have been developed to execute these algorithms with reduced cost and power consumption. The diverse range of specialized processors becoming available create great opportunities to deploy DNNs in new applications. But they also create challenges, as a DNN topology specifically designed for one processor may not run efficiently on a different processor. For developers of DNNs that run on multiple processor targets, the effort required to optimize the DNN for each processor can be prohibitive. In this talk, Woonhyun Nam, Algorithms Director at StradVision, explains cost-effective techniques that transform DNN layers to other layer types to better fit a specific processor, without the need to retrain from scratch. He also presents quantization and structured sparsification techniques which reduce model size and computation significantly. Nam discusses several case studies in the context of object detection and segmentation.
Joint Regularization of Activations and Weights for Efficient Neural Network Pruning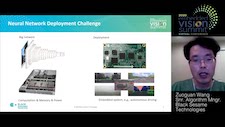
With the rapid increase in the sizes of deep neural networks (DNNs), there has been extensive research on network model compression to improve deployment efficiency. In this presentation, Zuoguan Wang, Senior Algorithm Manager at Black Sesame Technologies, presents his company’s work to advance compression beyond the weights to neuron activations. He proposes a joint regularization technique that simultaneously regulates the distribution of weights and activations. By distinguishing and leveraging the significant difference among neuron responses and connections during learning, the jointly pruned networks (JPnet) optimize the sparsity of activations and weights. The derived deep sparsification reveals more optimization space for existing DNN accelerators utilizing sparse matrix operations. Wang evaluates the effectiveness of joint regularization through various network models with different activation functions and on different datasets. With a 0.4% degradation constraint on inference accuracy, a JPnet can save 72% to 99% of computation cost, with up to 5.2x and 12.3x reductions in activation and weight numbers, respectively.
|
SENSING FUNDAMENTALS AND ADVANCEMENTS |
CMOS Image Sensors: A Guide to Building the Eyes of a Vision System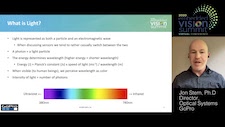
Improvements in CMOS image sensors have been instrumental in lowering barriers for embedding vision into a broad range of systems. For example, a high degree of system-on-chip integration allows photons to be converted into bits with minimal support circuitry. Low power consumption enables imaging in even small, battery-powered devices. Simple control protocols mean that companies can design camera-based systems without extensive in-house expertise. Meanwhile, the low cost of CMOS sensors is enabling visual perception to become ever more pervasive. In this tutorial, Jon Stern, Director of Optical Systems at GoPro, introduces the basic operation, types and characteristics of CMOS image sensors; explains how to select the right sensor for your application; and provides practical guidelines for building a camera module by pairing the sensor with suitable optics. He highlights areas demanding of special attention to equip you with an understanding of the common pitfalls in designing imaging systems.
Structures as Sensors: Smaller-Data Learning in the Physical World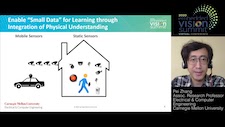
Machine learning has become a useful tool for many data-rich problems. However, its use in cyber-physical systems has been limited because of its need for large amounts of well-labeled data that must be tailored for each deployment, and the large number of variables that can affect data in the physical space (e.g., weather, time). This talk from Pei Zhang, Associate Research Professor at Carnegie Mellon University, introduces the problem through the concept of Structures as Sensors (SaS), in which the infrastructure (e.g., a building, or vehicle fleets) acts as the physical elements of the sensor, and the response is interpreted to obtain information about the occupants and about the environment. Zhang presents three physical-based approaches to reduce the data demand for robust learning in SaS:
- Generate data through the use of physical models,
- Improve sensed data through actuation of the sensing system, and
- Combine and transfer data from multiple deployments using physical understanding.
|
UPCOMING INDUSTRY EVENTS |
Securing Smart Devices: Protecting AI Models at the Edge – Sequitur Labs Webinar: September 28, 2021, 9:00 am PT
How Battery-powered Intelligent Vision is Bringing AI to the IoT – Eta Compute Webinar: October 5, 2021, 9:00 am PT
More Events
|
FEATURED NEWS |
Maxim Integrated’s Hand-held Camera Cube Reference Design Enables AI at the Edge for Vision and Hearing Applications
STMicroelectronics Latest STM32Cube.AI Release Strengthens Support for Efficient Machine Learning
NVIDIA’s v8 TensorRT AI Software Development Suite Delivers Inference Breakthroughs
Lattice Semiconductor’s Automate Solution Stack Accelerates the Development of Industrial Automation Systems
Renesas Electronics’ Entry-level RZ/V2L MPUs Supply High Power Efficiency and a High-precision AI Accelerator
More News
|
EDGE AI AND
VISION PRODUCT OF THE YEAR WINNER SHOWCASE |
Edge Impulse EON Compiler (Best Edge AI Developer Tool)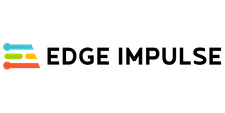
Edge Impulse’s EON Compiler is the 2021 Edge AI and Vision Product of the Year Award Winner in the Edge AI Developer Tools category. The Edge Impulse EON compiler accelerates embedded machine learning (ML) code to run faster on your neural network in 25-55% less RAM and up to 35% less flash memory, while retaining the same accuracy, compared to TensorFlow Lite for Microcontrollers. EON achieves this performance by compiling neural networks to C++, unlike other embedded solutions using generic interpreters, thus eliminating complex code, device power, and precious time. The EON compiler represents the new standard for tinyML developers seeking to bring better-embedded technologies to the market.
Please see here for more information on Edge Impulse’s EON Compiler. The Edge AI and Vision Product of the Year Awards celebrate the innovation of the industry’s leading companies that are developing and enabling the next generation of edge AI and computer vision products. Winning a Product of the Year award recognizes a company’s leadership in edge AI and computer vision as evaluated by independent industry experts. |