Dear Colleague,
Next Thursday, March 2, 2023 at 9 am PT, the Yole Group will deliver the free webinar “Short-wave Infrared: The Dawn of a New Imaging Age?” in partnership with the Edge AI and Vision Alliance. The term short wave refers to a portion of the infrared spectrum between 1 µm and 3 µm in wavelength. Short-wave infrared (SWIR) imagers based on InGaAs technology have found use in defense markets, for example, where they implement laser target designation and enhanced vision in harsh conditions, as well as in industrial applications such as semiconductor defect detection, package content inspection and inventory sorting.
Historically, SWIR imaging has been a niche market, with cost a key factor limiting broader adoption. InGaAs image sensors typically range in cost from a few thousand to more than ten thousand dollars. However, SWIR is now at a turning point, driven by the emergence of more cost-effective implementations based on quantum dots, germanium and various organic and other materials. The promise of lower-price image sensors has attracted interest in SWIR from consumer electronics and other high-volume applications. For this market growth potential to become a reality, however, the technology will also require further refinement in sensitivity, dark current, response time and other metrics.
In this webinar, Axel Clouet, Ph.D., technology and market analyst at the Yole Group, will discuss the history, current status and future technology and market trends for SWIR imaging, including comparisons with alternative imaging approaches, as well as the evolving and maturing ecosystem that supports SWIR imaging. A question-and-answer session will follow the presentation. For more information and to register, please see the event page.
Need to see the latest edge AI and vision processors, tools and algorithms? Looking for direct access to experts on visual and perceptual AI? Want to be the first to see the latest innovations and trends? If so, you should be at the 2023 Embedded Vision Summit, happening May 22-25 in Santa Clara, California.
The Summit is the event for practical computer vision and edge AI; you don’t want to miss it! Register now using discount code SUMMIT23-NL and you can save 25%. Don’t delay!
Brian Dipert
Editor-In-Chief, Edge AI and Vision Alliance |
How Transformers are Changing the Direction of Deep Learning Architectures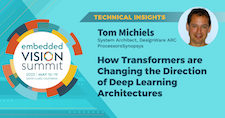
The neural network architectures used in embedded real-time applications are evolving quickly. Transformers are a leading deep learning approach for natural language processing and other time-dependent, series data applications. Now, transformer-based deep learning network architectures are also being applied to vision applications with state-of-the-art results compared to CNN-based solutions. In this presentation, Tom Michiels, System Architect for DesignWare ARC Processors at Synopsys, introduces transformers and contrasts them with the CNNs commonly used for vision tasks today. He examines the key features of transformer model architectures and shows performance comparisons between transformers and CNNs. He concludes the presentation with insights on why Synopsys thinks transformers are an important approach for future visual perception tasks.
The Nested Hierarchical Transformer: Towards Accurate, Data-efficient and Interpretable Visual Understanding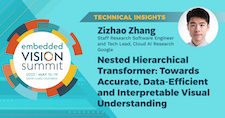
In computer vision, hierarchical structures are popular in vision transformers (ViT). In this talk, Zizhao Zhang, Staff Research Software Engineer and Tech Lead for Cloud AI Research at Google, presents a novel idea of nesting canonical local transformers on non-overlapping image blocks and aggregating them hierarchically. This new design, named NesT, leads to a simplified architecture compared with existing hierarchical structured designs, and requires only minor code changes relative to the original ViT. The benefits of the proposed judiciously-selected design are threefold:
- NesT converges faster and requires much less training data to achieve good generalization on both ImageNet and small datasets
- When extending key ideas to image generation, NesT leads to a strong decoder that is 8X faster than previous transformer-based generators, and
- Decoupling the feature learning and abstraction processes via the nested hierarchy in our design enables constructing a novel method (named GradCAT) for visually interpreting the learned model.
|
Comparing ML-based Audio with ML-based Vision: An Introduction to ML Audio for ML Vision Engineers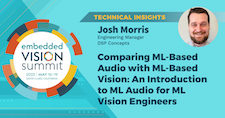
As embedded processors become more powerful, our ability to implement complex machine learning solutions at the edge is growing. Vision has led the way, solving problems as far-reaching as facial recognition and autonomous navigation. Now, ML audio is starting to appear in more and more edge applications, for example in the form of voice assistants, voice user interfaces and voice communication systems. Although audio data is quite different from video and image data, ML audio solutions often use many of the same techniques initially developed for video and images. In this talk, Josh Morris, Engineering Manager at DSP Concepts, introduces the ML techniques commonly used for audio at the edge, and compares and contrasts them with those commonly used for vision. After watching the video, you’ll be inspired to integrate ML-based audio into your next solution.
System Imperatives for Audio and Video AI at the Edge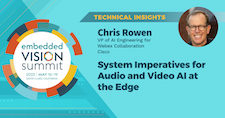
At long last, we are past the hype stage for media AI. Audio and video machine learning are becoming common tools for embedded hardware and software engineers. But are designers really using ML right? Are architects intelligently partitioning ML solutions between the cloud, user edge platforms and embedded compute components? Do they understand how to effectively combine deep-learning-based and conventional audio and video algorithms? Are they creating interfaces that enable their products to evolve in response to market needs? In this presentation, Chris Rowen, VP of AI Engineering for Webex Collaboration at Cisco, explores the conflicting currents pushing ML to the cloud and to the edge. He examines how the challenges of power, cost, compute, memory footprint, security and application autonomy affect different classes of audio and video devices and systems. He outlines strategies for teams planning new ML hardware and software to avoid some of the critical pitfalls and achieve better balance among time-to-market, application flexibility and system efficiency.
|
Edge Impulse EON Tuner (Best Edge AI Developer Tool)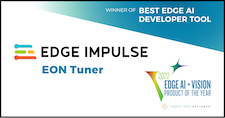
Edge Impulse’s EON Tuner was the 2022 Edge AI and Vision Product of the Year Award winner in the Edge AI Developer Tools category. The EON Tuner helps you find and select the best edge machine learning model for your application within the constraints of your target device. While existing “AutoML” tools focus only on machine learning, the EON Tuner performs end-to-end optimizations, from the digital signal processing (DSP) algorithm to the machine learning model, helping developers find and select the ideal tradeoff between these two types of processing blocks to achieve optimal performance for their computer vision application within the latency and memory constraints of their target edge device. The EON Tuner is designed to quickly assist developers in discovering preprocessing algorithms and NN model architectures specifically tailored for their use case and dataset. The EON Tuner eliminates the need for processing block and manual parameter selection to obtain the best model accuracy, reducing user’s technical knowledge requirements and decreasing the total time to get from data collection to a model that runs optimally on an edge device in the field.
Please see here for more information on Edge Impulse’s EON Tuner. The Edge AI and Vision Product of the Year Awards celebrate the innovation of the industry’s leading companies that are developing and enabling the next generation of edge AI and computer vision products. Winning a Product of the Year award recognizes a company’s leadership in edge AI and computer vision as evaluated by independent industry experts. |