LETTER FROM THE EDITOR |
Dear Colleague,
This Thursday, September 16 at 9 am PT, Edge Impulse will deliver the free webinar “Introducing the EON Tuner: Edge Impulse’s New AutoML Tool for Embedded Machine Learning” in partnership with the Edge AI and Vision Alliance. Finding the best machine learning (ML) model for analyzing sensor data isn’t easy. What pre-processing steps yield the best results, for example? And what signal processing parameters should you pick? The selection process is even more challenging when the resulting model needs to run on a microcontroller with significant latency, memory and power constraints. AutoML tools can help, but typically only look at the neural network, disregarding the important roles that pre-processing and signal processing play with tinyML. This hands-on session will introduce the EON Tuner, a new AutoML tool available to all Edge Impulse developers. You’ll learn how to use EON Tuner to pick the optimum model within the constraints of your device, improving the accuracy of your audio, computer vision and other sensor data classification projects. The webinar will include demonstrations of the concepts discussed. For more information and to register, please see the event page.
Brian Dipert
Editor-In-Chief, Edge AI and Vision Alliance |
DEEP LEARNING ADVANCEMENTS |
New Methods for Implementation of 2-D Convolution for Convolutional Neural Networks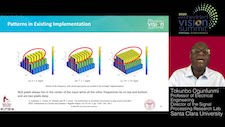
The increasing usage of convolutional neural networks (CNNs) in various applications on mobile and embedded devices and in data centers has led researchers to explore application specific hardware accelerators for CNNs. CNNs typically consist of a number of convolution, activation and pooling layers, with convolution layers being the most computationally demanding. Though popular for accelerating CNN training and inference, GPUs are not ideal for embedded applications because they are not energy efficient. ASIC and FPGA accelerators have the potential to run CNNs in a highly efficient manner. In this talk, Tokunbo Ogunfunmi, Professor of Electrical Engineering and Director of the Signal Processing Research Laboratory at Santa Clara University, presents two new methods for 2-D convolution that offer significant reduction in power consumption and computational complexity. The first method computes convolution results using row-wise inputs, as opposed to traditional tile-based processing, yielding considerably reduced latency. The second method, single partial product 2-D (SPP2D) convolution, avoids recalculation of partial weights and reduces input reuse. Hardware implementation results are presented.
Imaging Systems for Applied Reinforcement Learning Control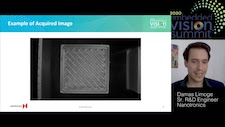
Reinforcement learning has generated human-level decision-making strategies in highly complex game scenarios. But most industries, such as manufacturing, have not seen impressive results from the application of these algorithms, belying the utility hoped for by their creators. The limitations of reinforcement learning in real use cases intuitively manifest from the number of exploration examples needed to train the underlying models, but also from incomplete state representations for an artificial agent to act on. In an effort to improve automated inspection for factory control through reinforcement learning, Nanotronics’ research is focused on improving the state representation of a manufacturing process using optical inspection as a basis for agent optimization. In this presentation, Damas Limoge, Senior R&D Engineer at Nanotronics, focuses on the imaging system: its design, implementation and utilization, in the context of a reinforcement agent.
|
EDGE DEVICE OPTIMIZATIONS |
Deep Learning on Mobile Devices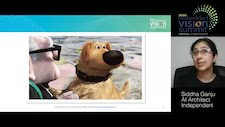
Over the last few years, convolutional neural networks (CNNs) have grown enormously in popularity, especially for computer vision. Many applications running on smartphones and wearable devices could benefit from the capabilities of CNNs. However, CNNs are by nature computation- and memory-intensive, making them challenging to deploy on a mobile device. In this presentation, independent AI architect Siddha Ganju shares easy-to-use, practical techniques that can improve CNN performance on mobile devices.
DevCloud for the Edge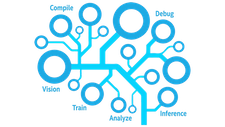
In this video, Jeff Bier, Founder of the Edge AI and Vision Alliance, interviews Monique Jones, Senior Software Engineer Team Lead at Intel, about her company’s DevCloud for the Edge developer toolset. Bier and Jones compare and contrast a DevCloud-based development flow versus the traditional approach that leverages a development board located at the engineer’s desk and directly connected to a computer, highlighting the advantages of the cloud-based approach and showcasing some of the toolset’s key features and capabilities. DevCloud for the Edge allows you to virtually prototype and experiment with AI workloads for computer vision on the latest Intel edge inferencing hardware, with no hardware setup required since the code executes directly within the web browser. You can test the performance of your models using the Intel Distribution of OpenVINO Toolkit and combinations of CPUs, GPUs, VPUs and FPGAs. The site also contains a series of tutorials and examples preloaded with everything needed to quickly get started, including trained models, sample data and executable code from the Intel Distribution of OpenVINO Toolkit as well as other deep learning tools.
|
UPCOMING INDUSTRY EVENTS |
Introducing the EON Tuner: Edge Impulse’s New AutoML Tool for Embedded Machine Learning – Edge Impulse Webinar: September 16, 2021, 9:00 am PT
Securing Smart Devices: Protecting AI Models at the Edge – Sequitur Labs Webinar: September 28, 2021, 9:00 am PT
How Battery-powered Intelligent Vision is Bringing AI to the IoT – Eta Compute Webinar: October 5, 2021, 9:00 am PT
More Events
|
FEATURED NEWS |
STMicroelectronics’ New 8×8 Multi-Zone Ranging Time-of-Flight Sensor Brings Distance Discernment to a Spectrum of Consumer and Industrial Products
MediaTek’s Latest AI-enhanced Application Processors Include the Kompanio 900T for Tablets and Notebooks and Kompanio 1300T for Premium Tablets
Efinix Has Announced AEC-Q100 Qualification and an Automotive Product Line Initiative for Its Programmable Logic Devices
Ambarella’s Partnerships with KeepTruckin and Yandex Target Vehicle Fleet Applications for Computer Vision
Unikie and Ericsson’s Combined Development Leverages Visual AI and a 5G Network for Real-time Remote Vehicle Steering
More News
|
EDGE AI AND VISION PRODUCT OF THE YEAR WINNER SHOWCASE |
Simbe Robotics Tally 3.0 (Best Enterprise Edge AI End Product)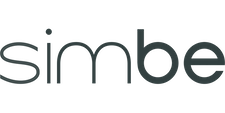
Simbe Robotics’ Tally 3.0 is the 2021 Edge AI and Vision Product of the Year Award Winner in the Enterprise Edge AI End Products category. Tally is the only robot on the market that combines computer vision, machine learning, and RFID technologies to audit store shelves across a range of retail environments. Tally detects misplaced, mispriced, and out-of-stock items, arming retailers with stronger insights into shelf availability, and ensuring that items are more quickly restocked and corrected, improving the customer experience. Tally 3.0 combines both edge and cloud computing, enabling it to transfer some of its AI and machine learning workloads to the edge. This hybrid system better optimizes data collection and processing, getting insights to store teams more quickly. By operating both on the edge and in the cloud, Tally can more quickly use deep learning to help with tasks like autofocus and barcode decoding, ensuring stores have the most up-to-date data.
Please see here for more information on Simbe Robotics’ Tally 3.0. The Edge AI and Vision Product of the Year Awards celebrate the innovation of the industry’s leading companies that are developing and enabling the next generation of edge AI and computer vision products. Winning a Product of the Year award recognizes a company’s leadership in edge AI and computer vision as evaluated by independent industry experts. |