Tenyks is a University of Cambridge spin-out, backed by YCombinator, helping Machine Learning Teams build production-ready models 8x faster by detecting and removing hidden vulnerabilities, biases, and blindspots before something breaks, crashes, or burns.
Tenyks
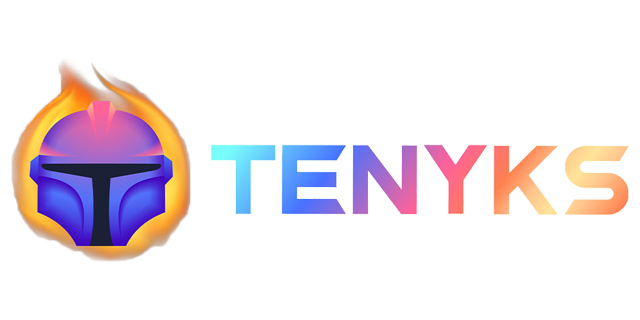
Recent Content by Company
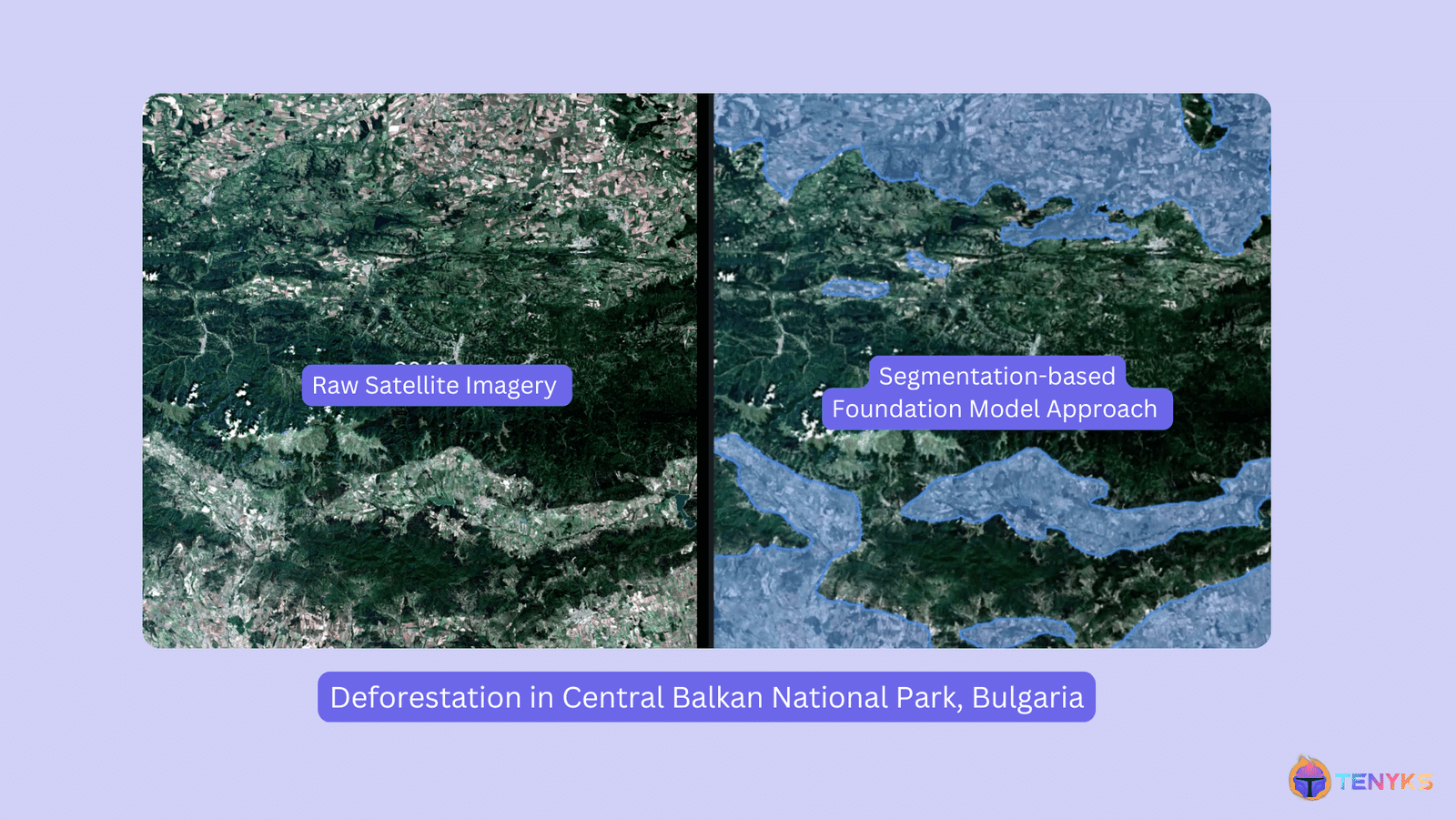
Visual Intelligence: Foundation Models + Satellite Analytics for Deforestation (Part 2)
This blog post was originally published at Tenyks’ website. It is reprinted here with the permission of Tenyks. In Part 2, we explore how Foundation Models can be leveraged to track deforestation patterns. Building upon the insights from our Sentinel-2 pipeline and Central Balkan case study, we dive into the revolution that foundation models have […]
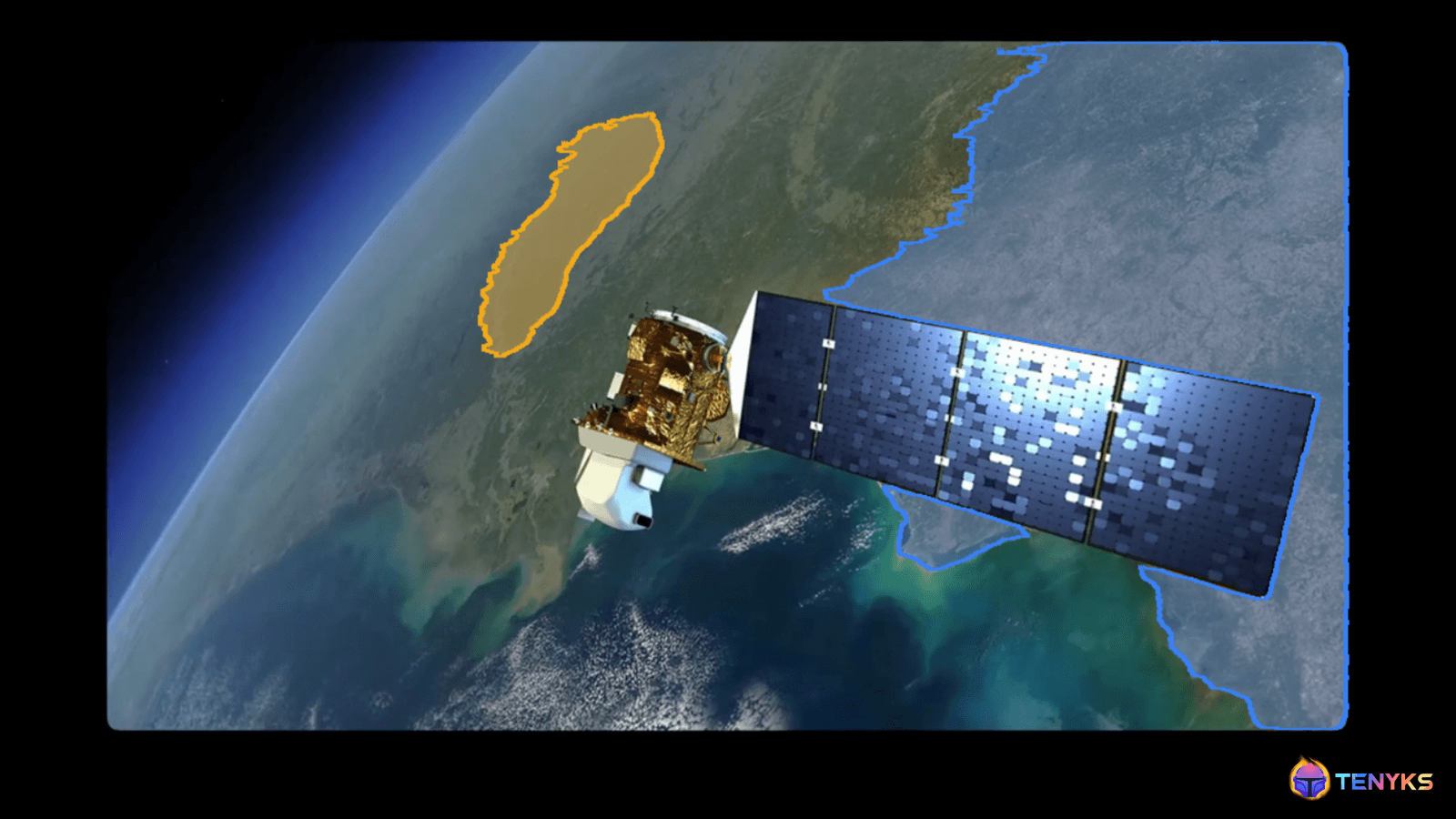
Visual Intelligence: Foundation Models + Satellite Analytics for Deforestation (Part 1)
This blog post was originally published at Tenyks’ website. It is reprinted here with the permission of Tenyks. Satellite imagery has revolutionized how we monitor Earth’s forests, offering unprecedented insights into deforestation patterns. In this two-part series, we explore both traditional and cutting-edge approaches to forest monitoring, using Bulgaria’s Central Balkan National Park as our […]
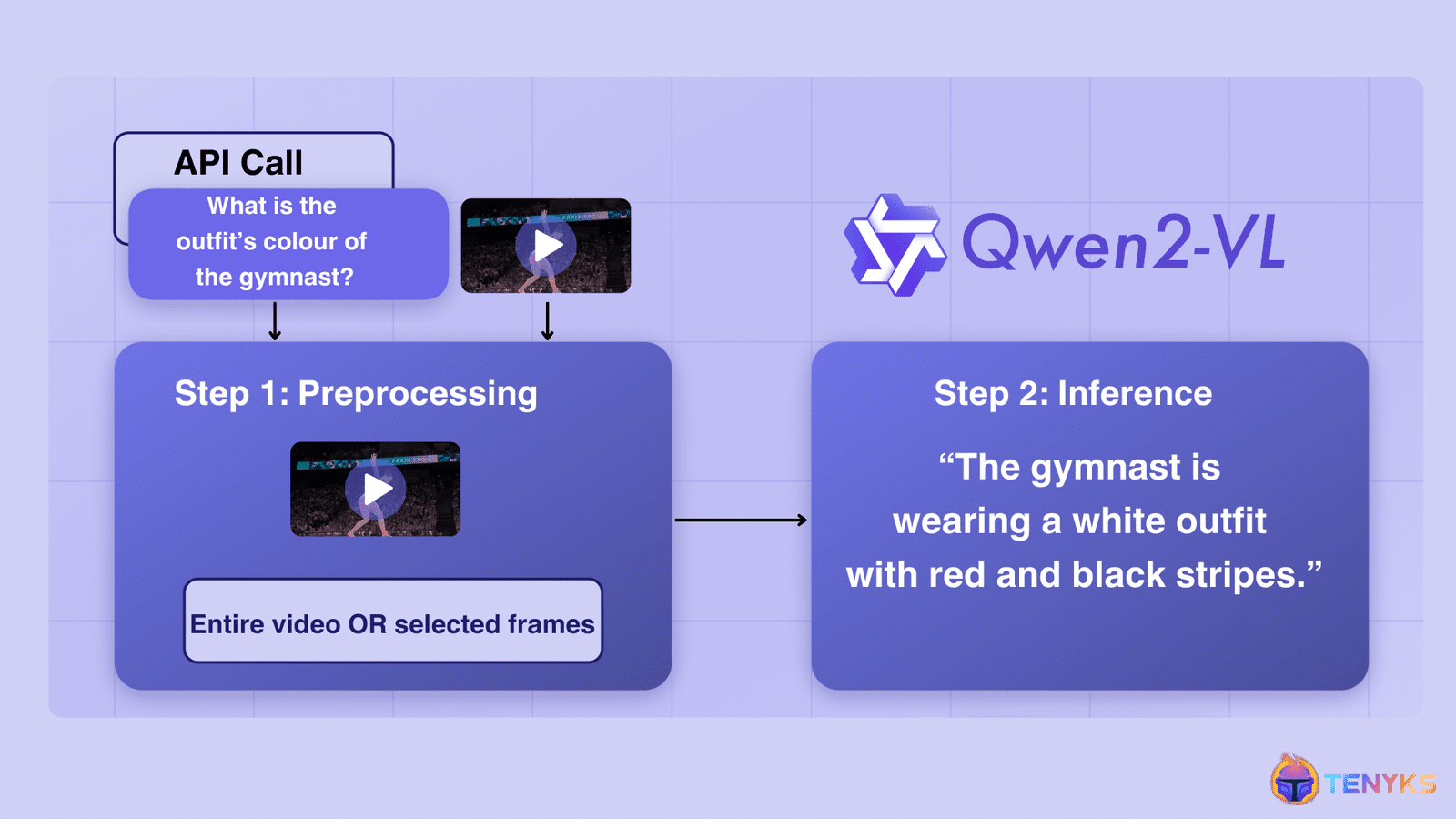
Video Understanding: Qwen2-VL, An Expert Vision-language Model
This article was originally published at Tenyks’ website. It is reprinted here with the permission of Tenyks. Qwen2-VL, an advanced vision language model built on Qwen2 [1], sets new benchmarks in image comprehension across varied resolutions and ratios, while also tackling extended video content. Though Qwen2-V excels at many fronts, this article explores the model’s […]
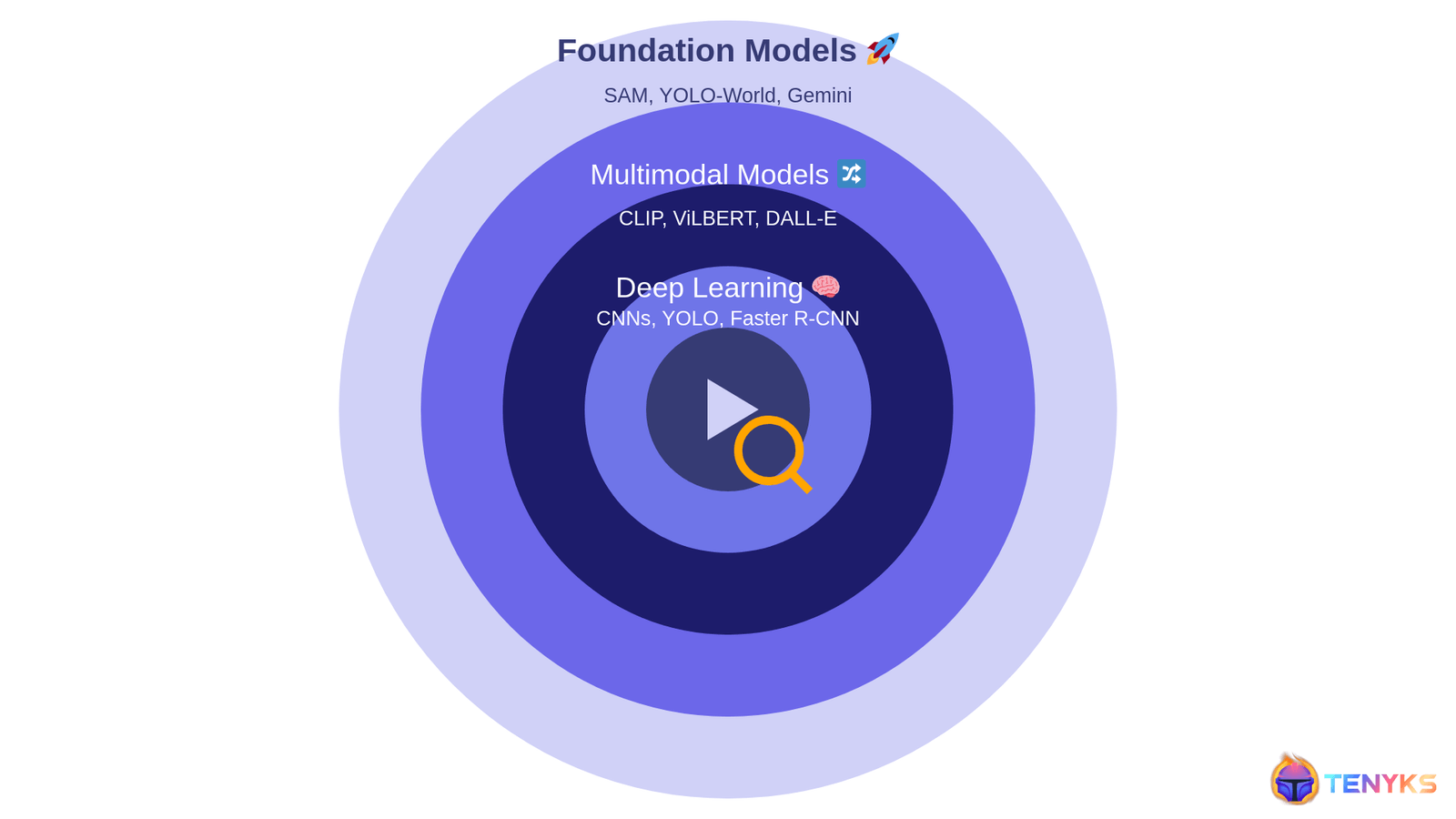
Scalable Video Search: Cascading Foundation Models
This article was originally published at Tenyks’ website. It is reprinted here with the permission of Tenyks. Video has become the lingua franca of the digital age, but its ubiquity presents a unique challenge: how do we efficiently extract meaningful information from this ocean of visual data? In Part 1 of this series, we navigate […]
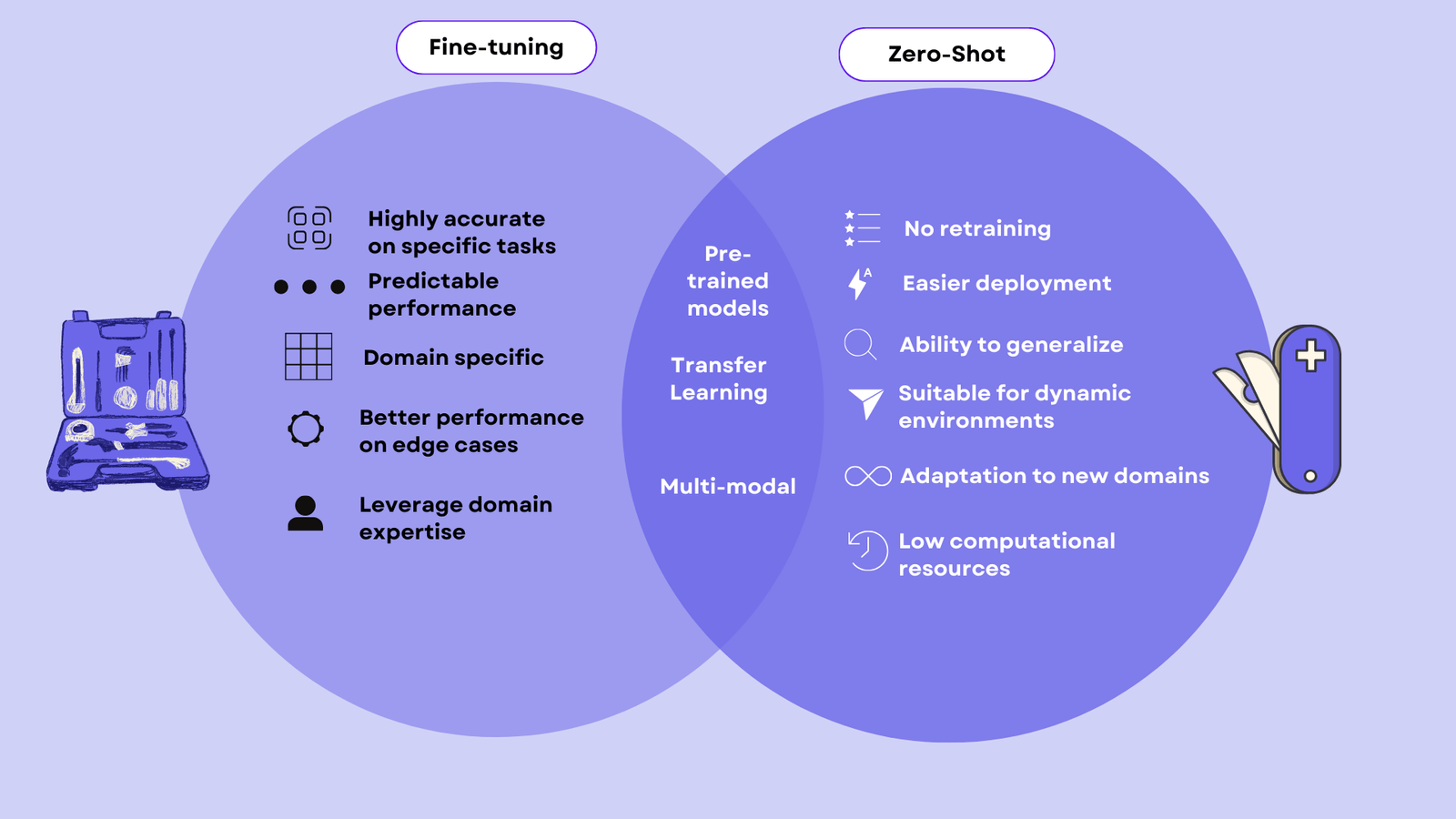
Zero-Shot AI: The End of Fine-tuning as We Know It?
This article was originally published at Tenyks’ website. It is reprinted here with the permission of Tenyks. Models like SAM 2, LLaVA or ChatGPT can do tasks without special training. This has people wondering if the old way (i.e., fine-tuning) of training AI is becoming outdated. In this article, we compare two models: YOLOv8 (fine-tuning) […]
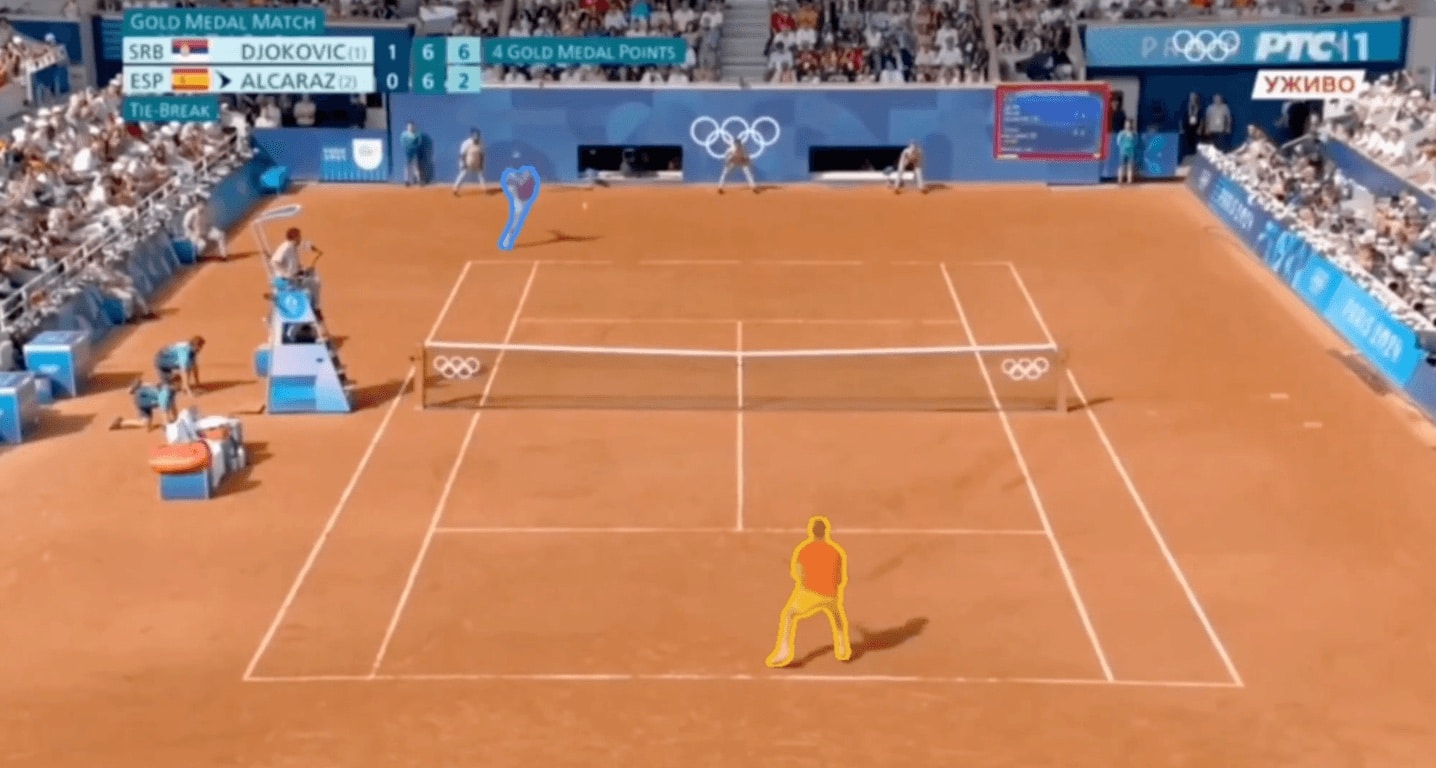
SAM 2 + GPT-4o: Cascading Foundation Models via Visual Prompting (Part 2)
This article was originally published at Tenyks’ website. It is reprinted here with the permission of Tenyks. In Part 2 of our Segment Anything Model 2 (SAM 2) Series, we show how foundation models (e.g., GPT-4o, Claude Sonnet 3.5 and YOLO-World) can be used to generate visual inputs (e.g., bounding boxes) for SAM 2. Learn […]
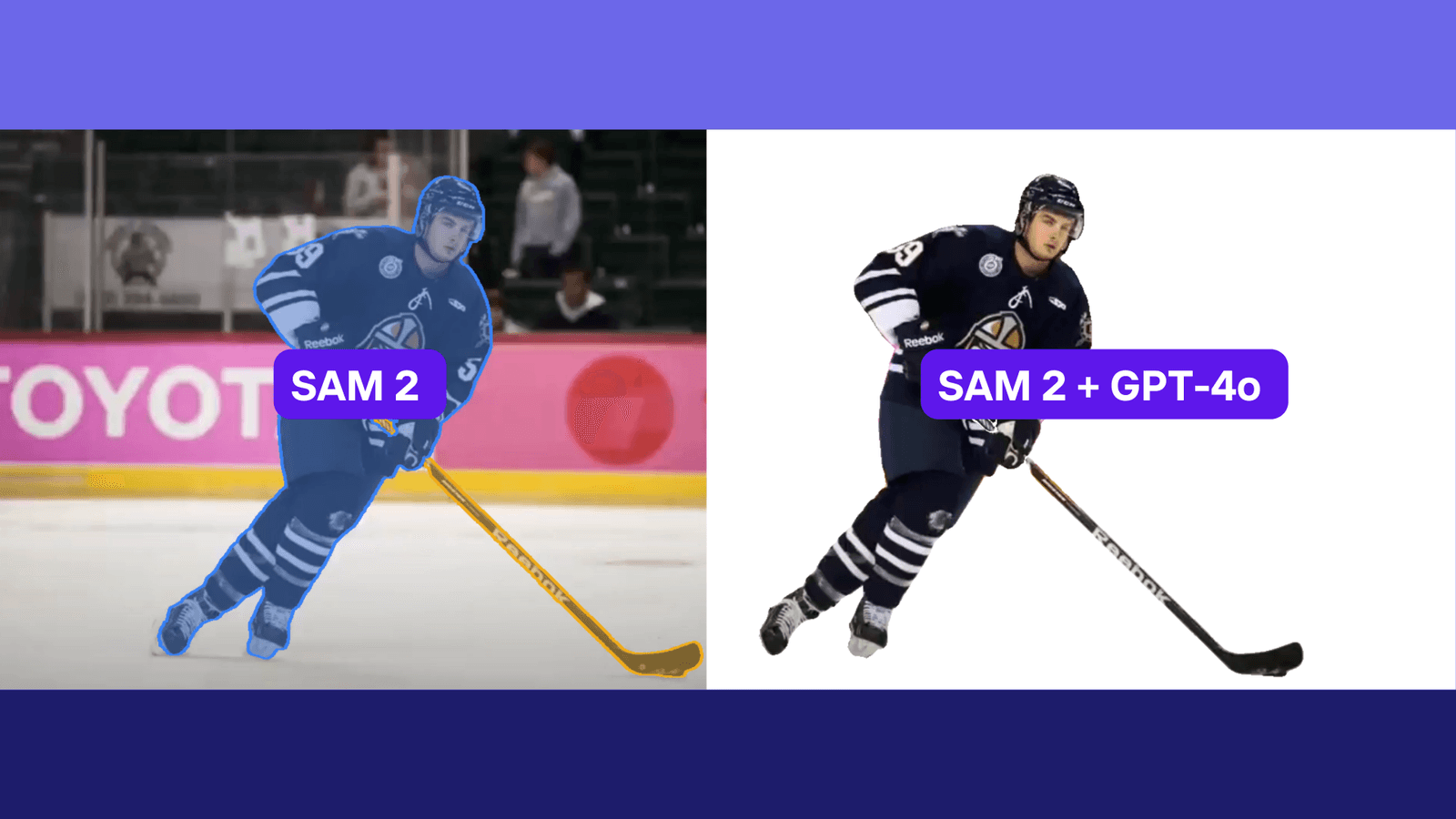
SAM 2 + GPT-4o: Cascading Foundation Models via Visual Prompting (Part 1)
This article was originally published at Tenyks’ website. It is reprinted here with the permission of Tenyks. In Part 1 of this article we introduce Segment Anything Model 2 (SAM 2). Then, we walk you through how you can set it up and run inference on your own video clips. Learn more about visual prompting […]
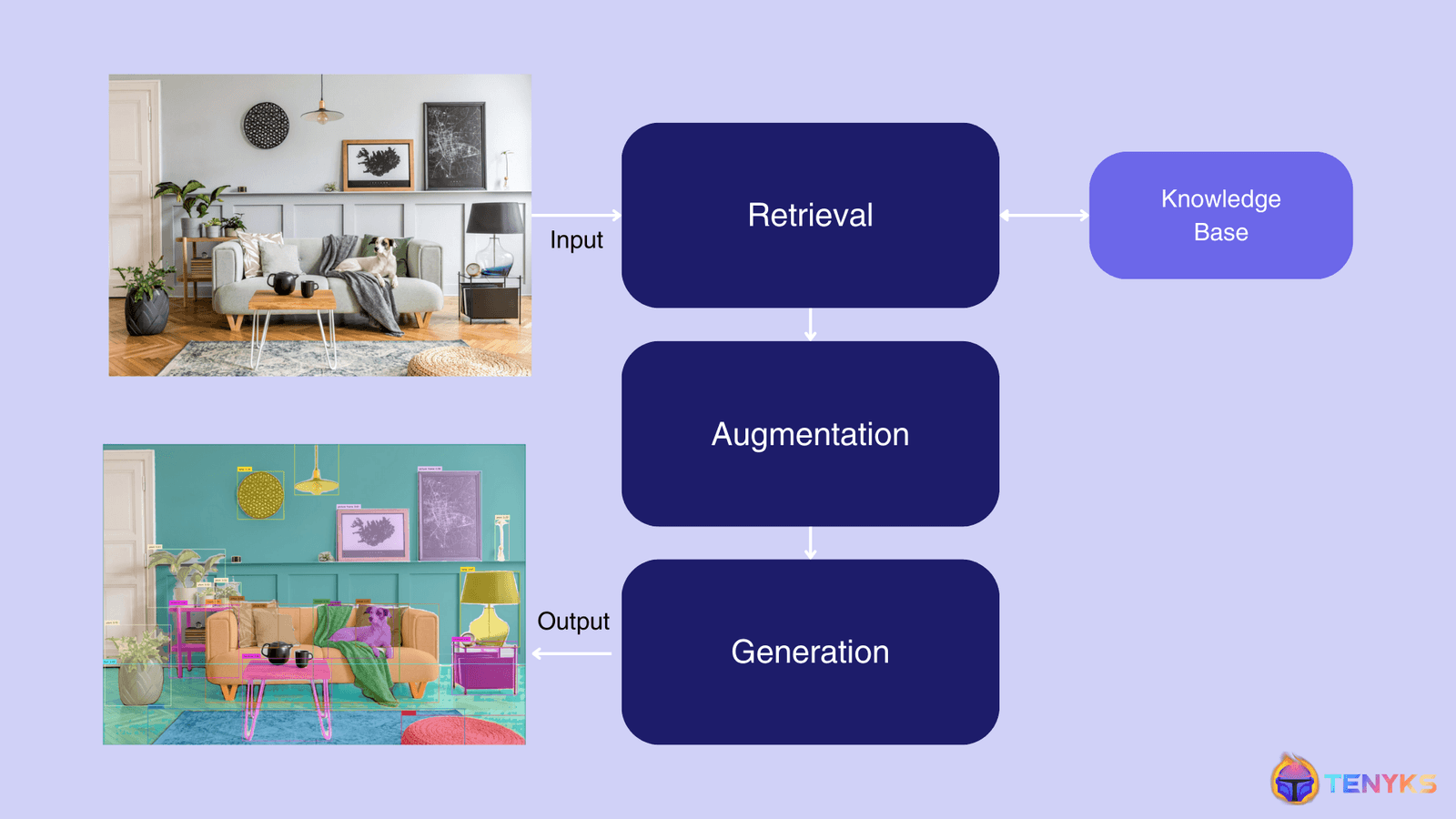
RAG for Vision: Building Multimodal Computer Vision Systems
This blog post was originally published at Tenyks’ website. It is reprinted here with the permission of Tenyks. This article explores the exciting world of Visual RAG, exploring its significance and how it’s revolutionizing traditional computer vision pipelines. From understanding the basics of RAG to its specific applications in visual tasks and surveillance, we’ll examine […]
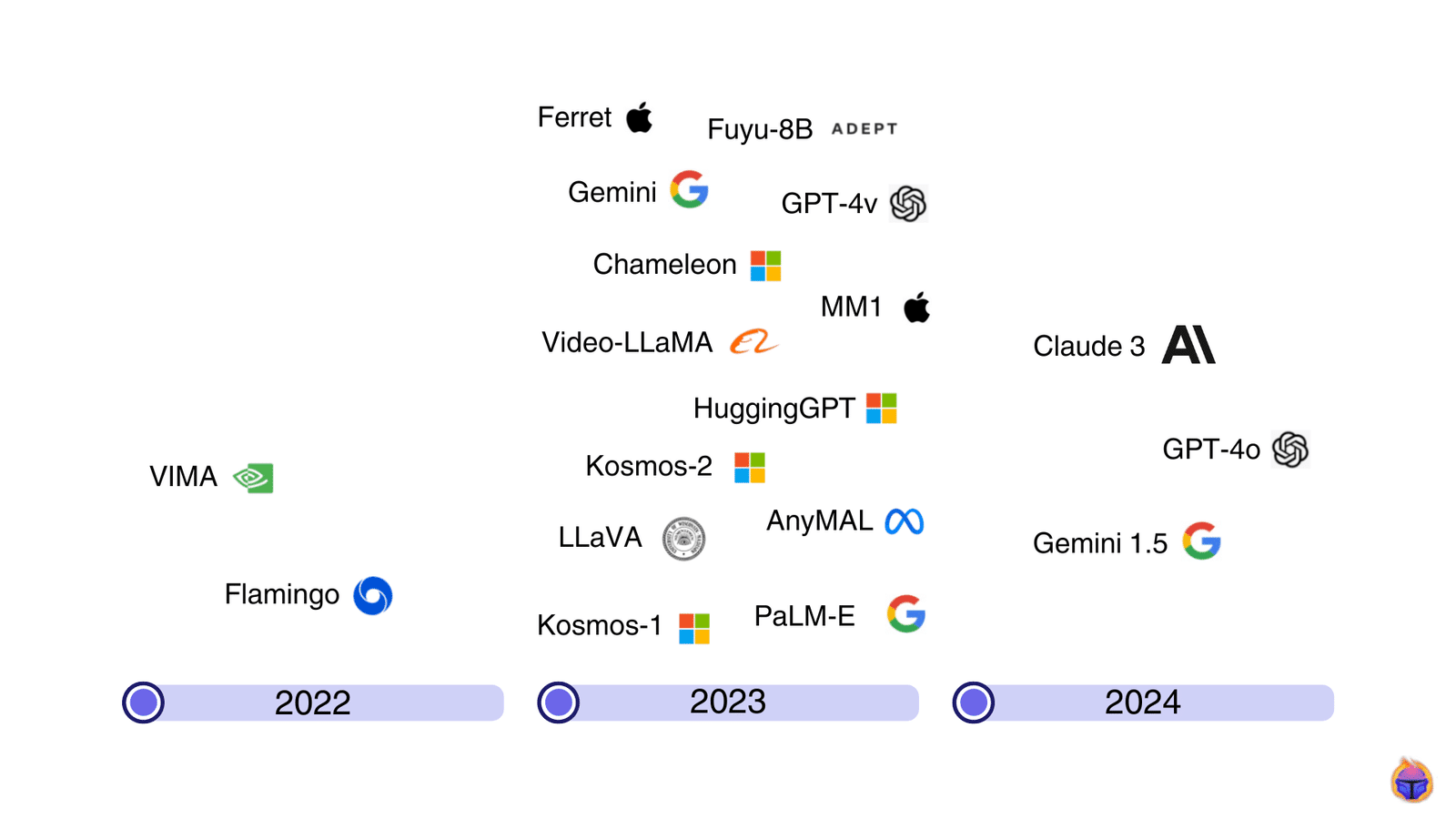
Multimodal Large Language Models: Transforming Computer Vision
This blog post was originally published at Tenyks’ website. It is reprinted here with the permission of Tenyks. This article introduces multimodal large language models (MLLMs) [1], their applications using challenging prompts, and the top models reshaping computer vision as we speak. What is a multimodal large language model (MLLM)? In layman terms, a multimodal […]
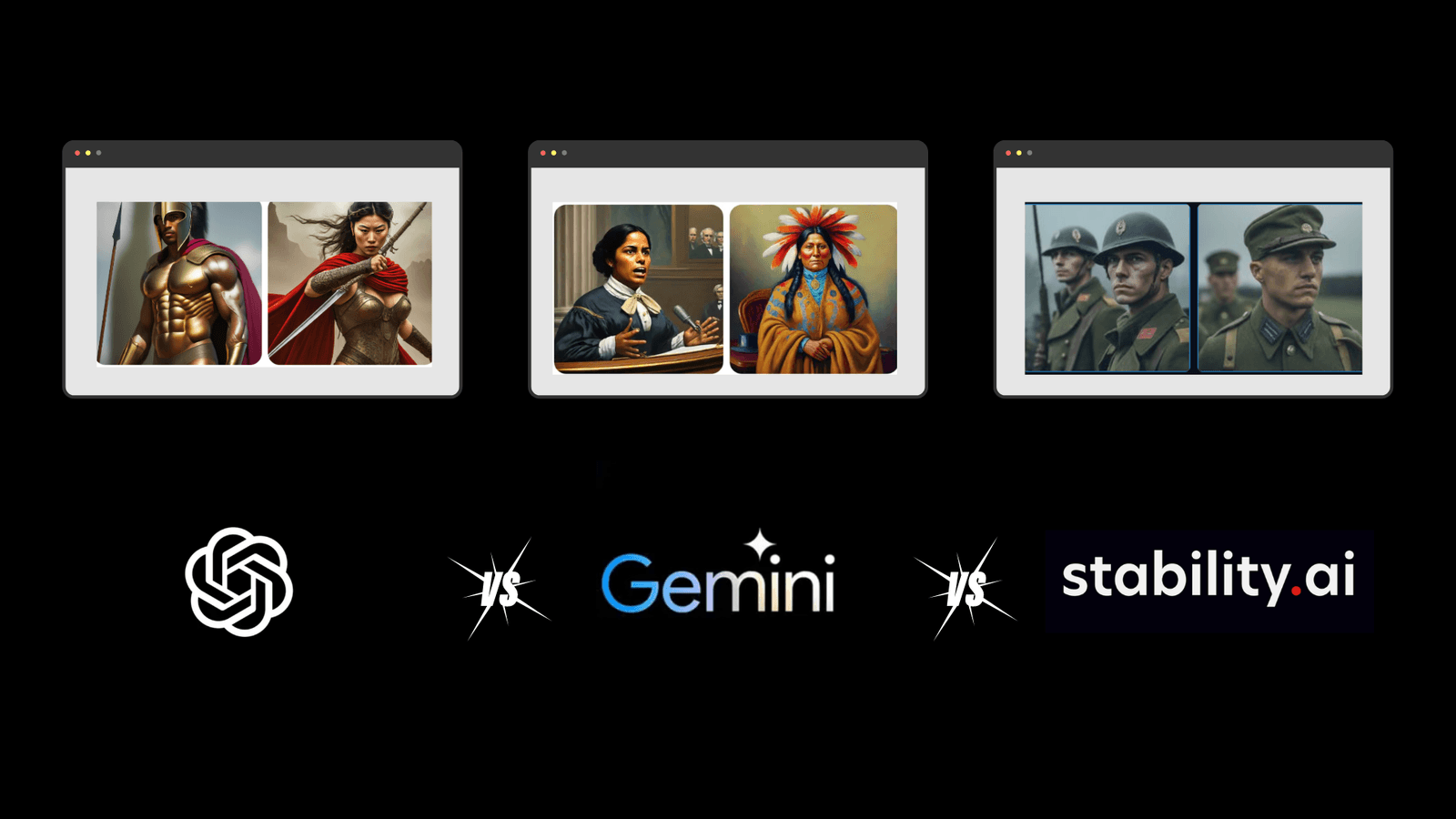
DALL-E vs Gemini vs Stability: GenAI Evaluations
This article was originally published at Tenyks’ website. It is reprinted here with the permission of Tenyks. We performed a side-by-side comparison of three models from leading providers in Generative AI for Vision. This is what we found: Despite the subjectivity involved in Human Evaluation, this is the best approach to evaluate state-of-the-art GenAI Vision […]
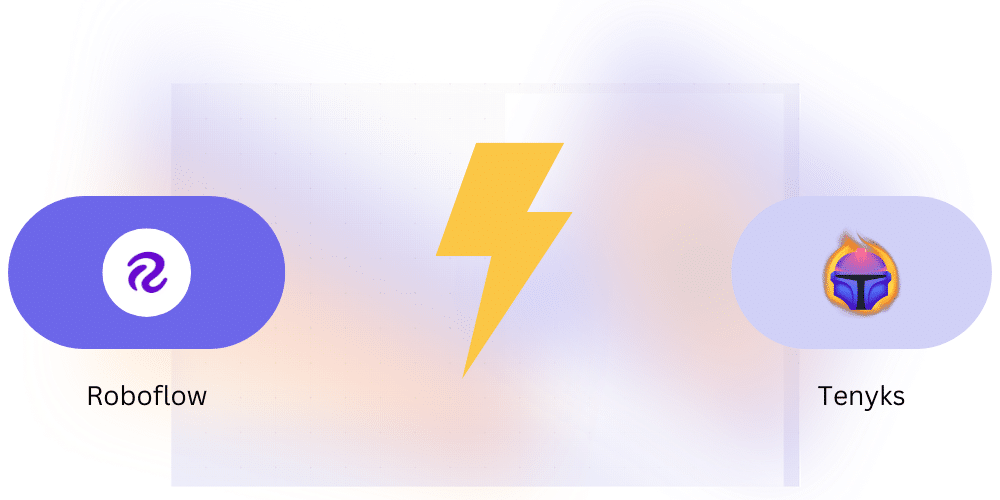
Improving Vision Model Performance Using Roboflow and Tenyks
This blog post was originally published at Tenyks’ website. It is reprinted here with the permission of Tenyks. When improving an object detection model, many engineers focus solely on tweaking the model architecture and hyperparameters. However, the root cause of mediocre performance often lies in the data itself. In this collaborative post between Roboflow and […]
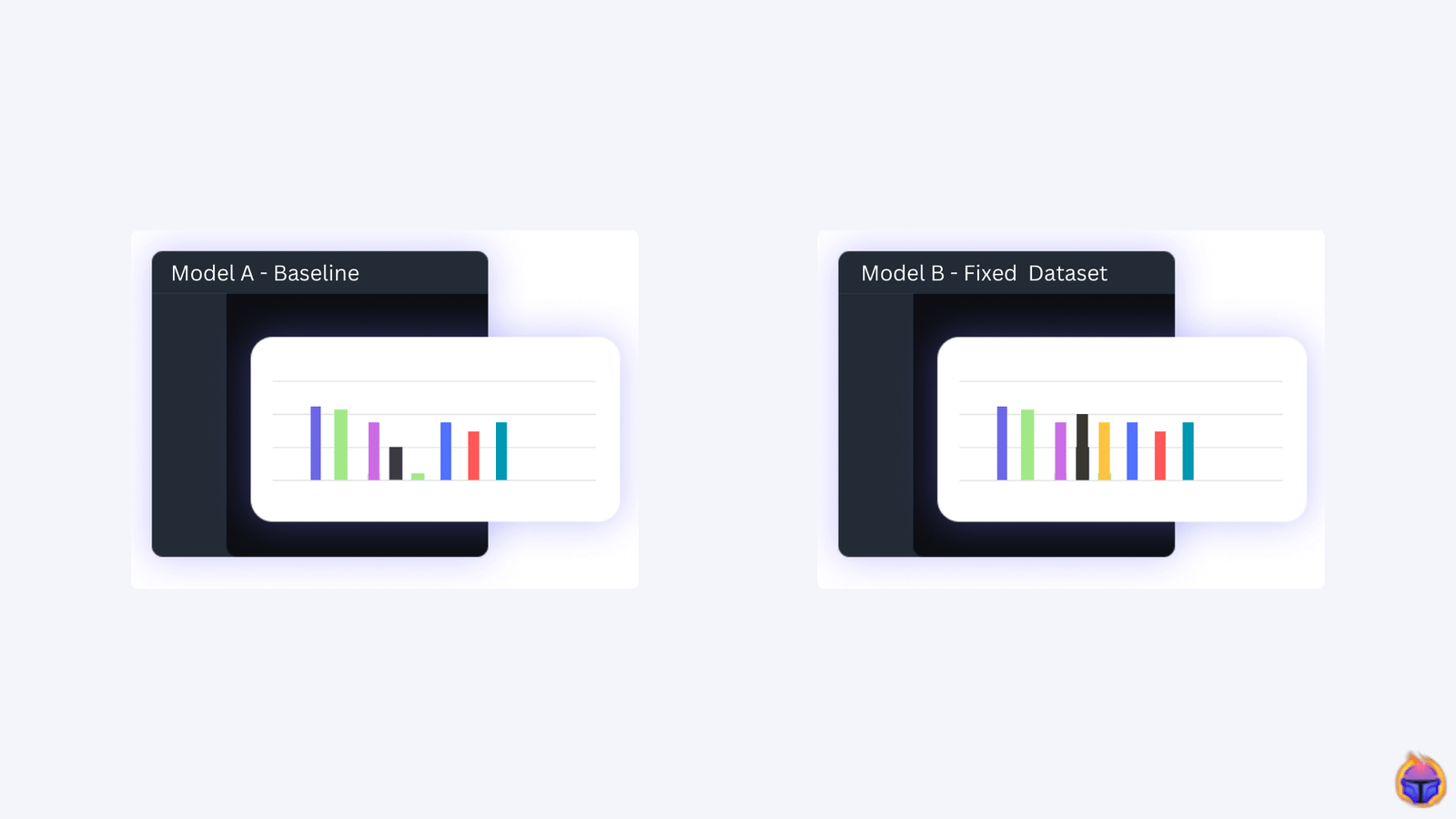
NVIDIA TAO Toolkit: How to Build a Data-centric Pipeline to Improve Model Performance (Part 3 of 3)
This blog post was originally published at Tenyks’ website. It is reprinted here with the permission of Tenyks. During this series, we will use Tenyks to build a data-centric pipeline to debug and fix a model trained with the NVIDIA TAO Toolkit. Part 1. We demystify the NVIDIA ecosystem and define a data-centric pipeline based […]
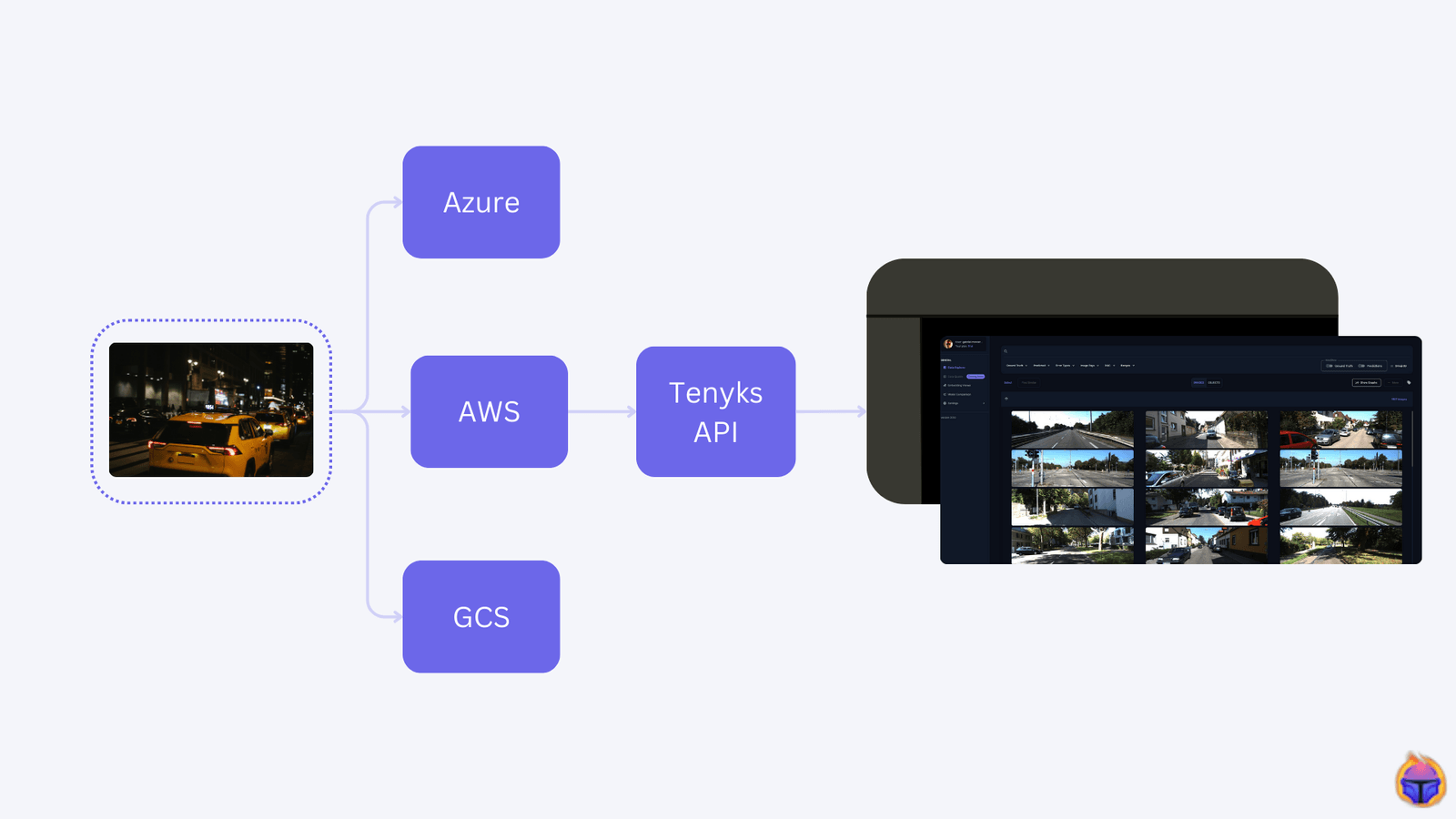
NVIDIA TAO Toolkit: How to Build a Data-centric Pipeline to Improve Model Performance (Part 2 of 3)
This blog post was originally published at Tenyks’ website. It is reprinted here with the permission of Tenyks. During this series, we will use Tenyks to build a data-centric pipeline to debug and fix a model trained with the NVIDIA TAO Toolkit. Part 1. We demystify the NVIDIA ecosystem and define a data-centric pipeline based […]
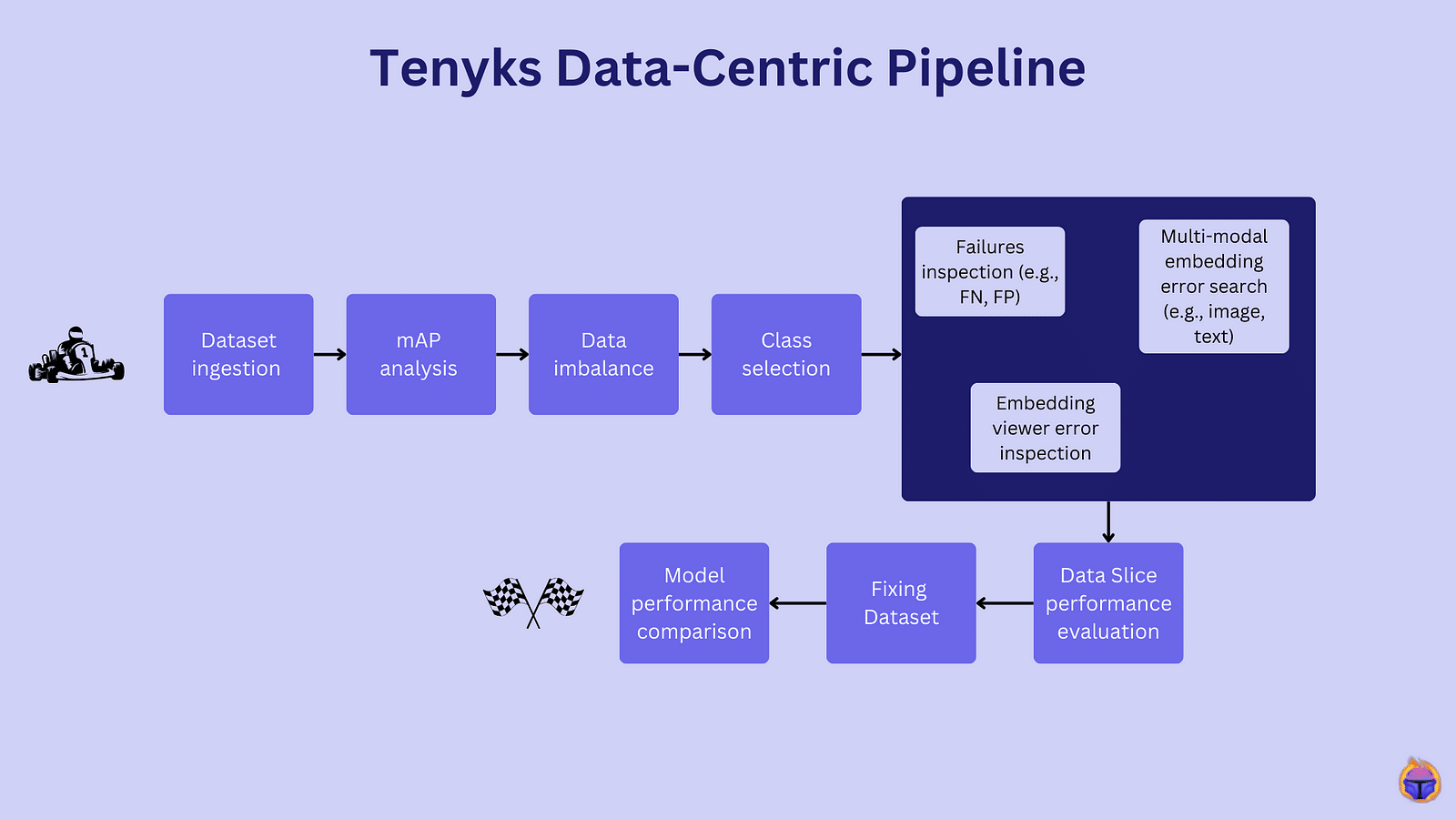
NVIDIA TAO Toolkit: How to Build a Data-centric Pipeline to Improve Model Performance (Part 1 of 3)
This blog post was originally published at Tenyks’ website. It is reprinted here with the permission of Tenyks. In this series, we’ll build a data-centric pipeline using Tenyks to debug and fix a model trained with the NVIDIA TAO Toolkit. Part 1. We demystify the NVIDIA ecosystem and define a data-centric pipeline tailored for a […]
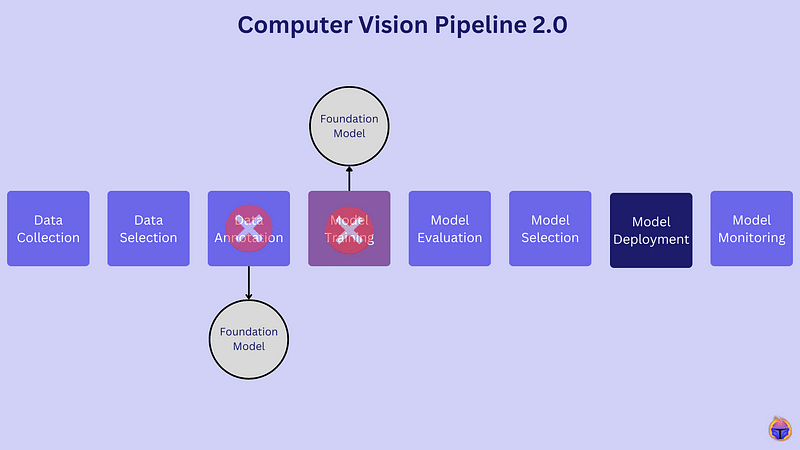
Computer Vision Pipeline v2.0
This blog post was originally published at Tenyks’ website. It is reprinted here with the permission of Tenyks. In the realm of computer vision, a shift is underway. This article explores the transformative power of foundation models, digging into their role in reshaping the entire computer vision pipeline. It also demystifies the hype behind the […]
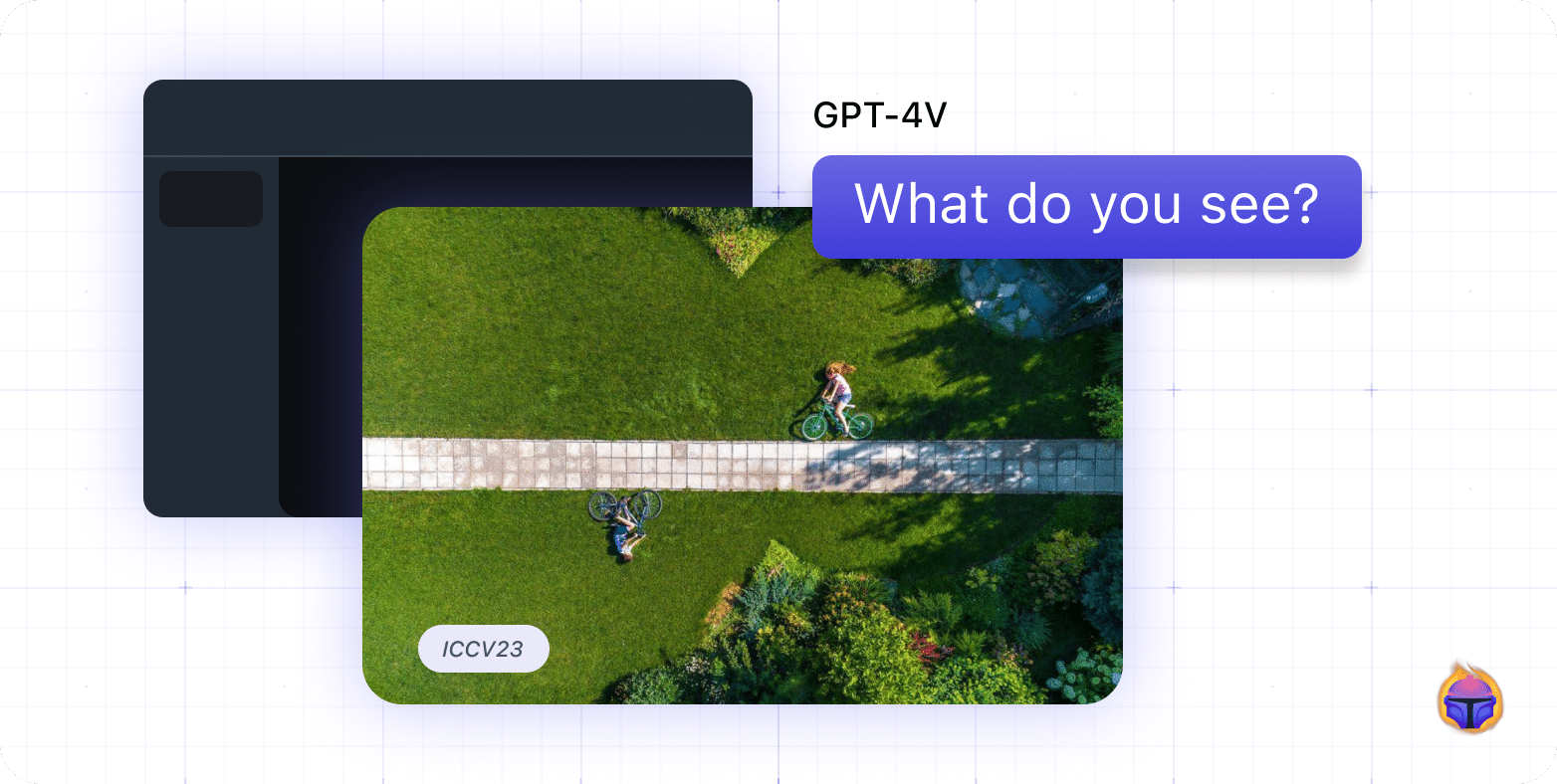
Amid the Rise of LLMs, is Computer Vision Dead?
This blog post was originally published at Tenyks’ website. It is reprinted here with the permission of Tenyks. The field of computer vision has seen incredible progress, but some believe there are signs it is stalling. At the International Conference on Computer Vision 2023 workshop “Quo Vadis, Computer Vision?”, researchers discussed what’s next for computer […]
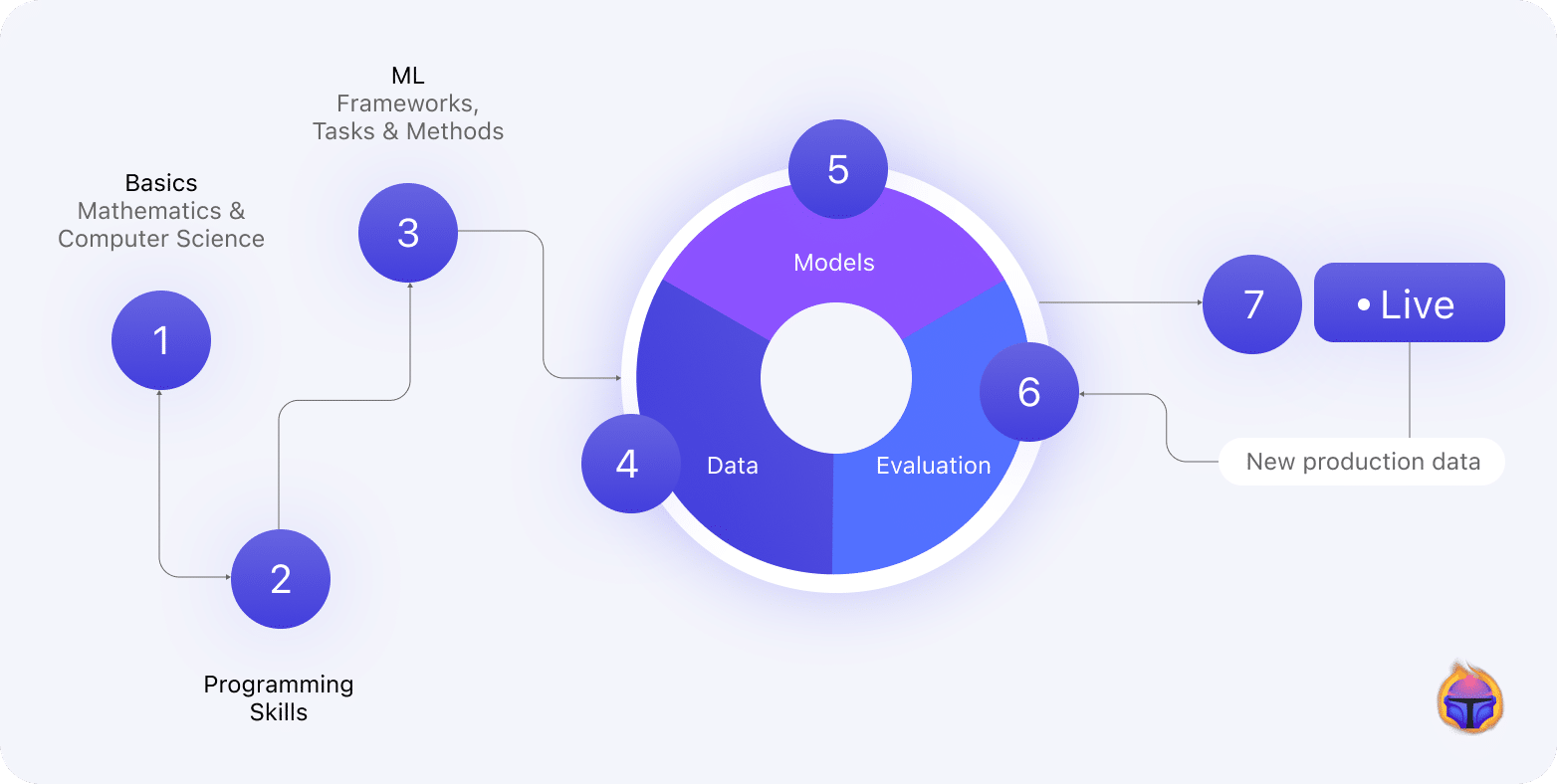
Becoming a Computer Vision Engineer
This blog post was originally published at Tenyks’ website. It is reprinted here with the permission of Tenyks. In the journey to become a proficient computer vision engineer, mastering the skills required at each stage of the machine learning life-cycle is crucial. This article introduces a blueprint with the skills a computer vision engineer is […]
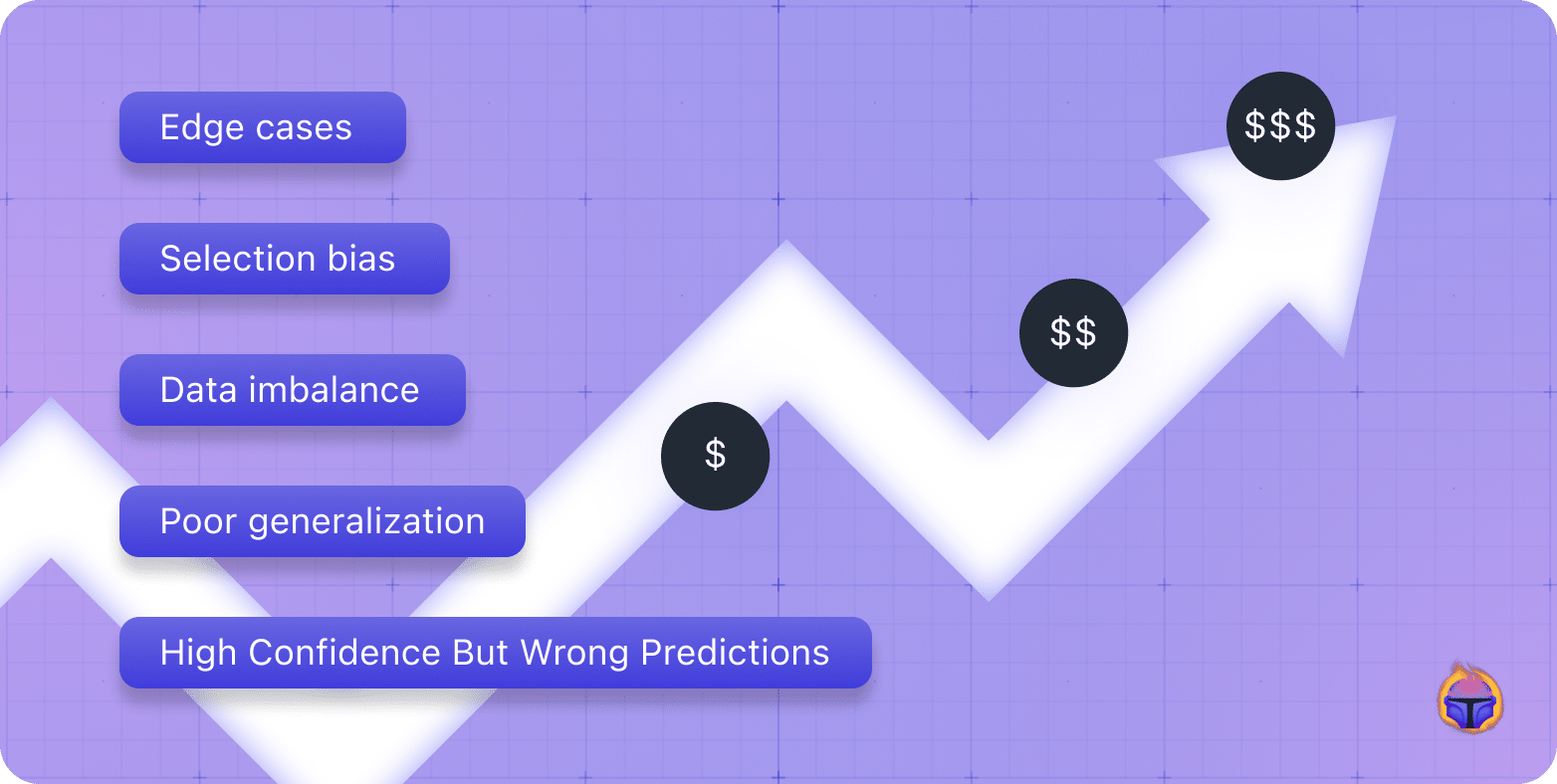
The Unseen Cost of Low Quality Large Datasets
This blog post was originally published at Tenyks’ website. It is reprinted here with the permission of Tenyks. Your current data selection process may be limiting your models. Massive datasets come with obvious storage and compute costs. But the two biggest challenges are often hidden: Money and Time. With increasing data volumes, companies have a […]
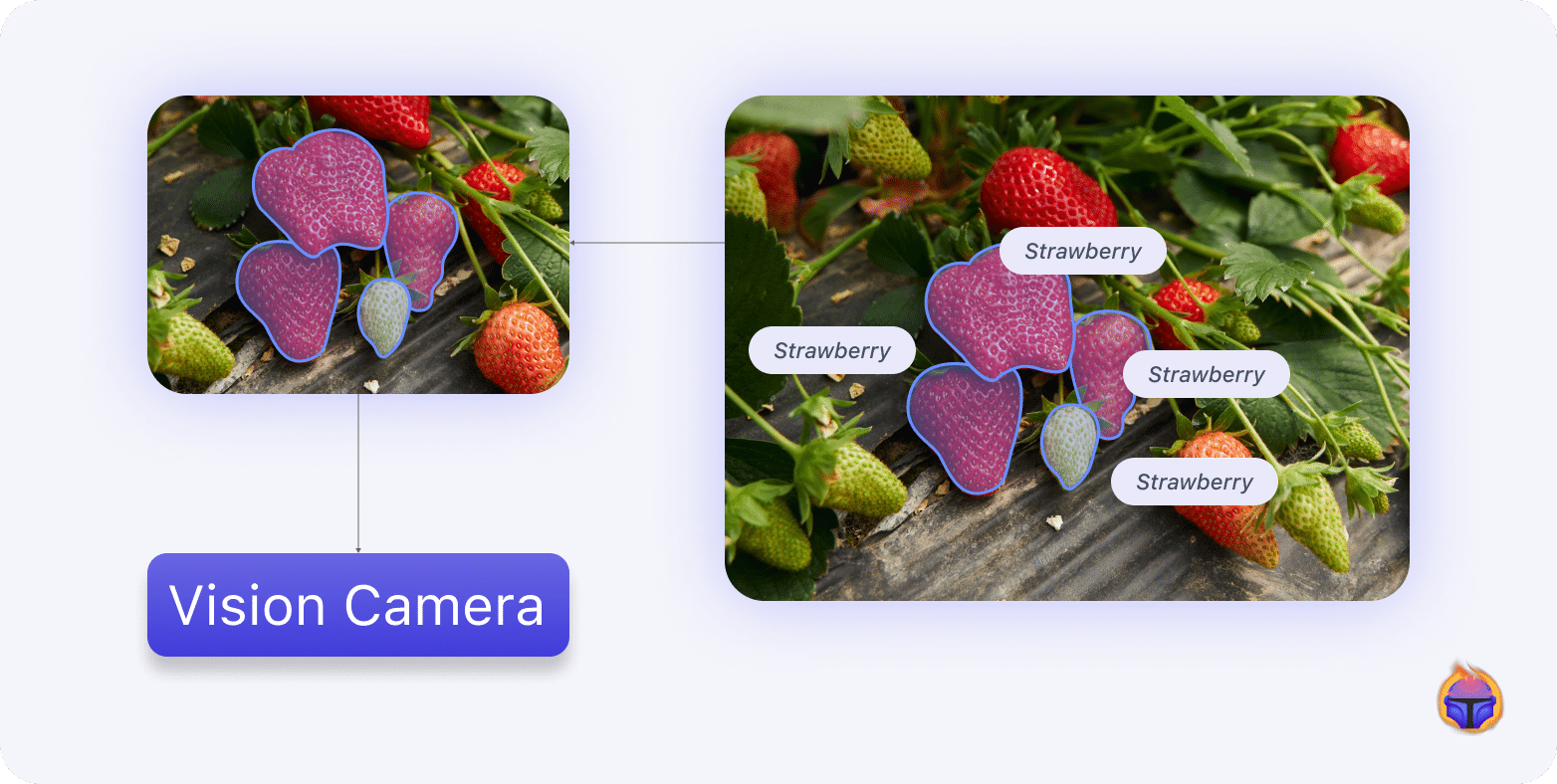
Top 4 Computer Vision Problems & Solutions in Agriculture — Part 2
This blog post was originally published at Tenyks’ website. It is reprinted here with the permission of Tenyks. In Part 1 of this series we introduced you with the top 4 issues you are likely to encounter in agriculture related datasets for object detection: occlusion, label quality, data imbalance and scale variation. In Part 2 […]
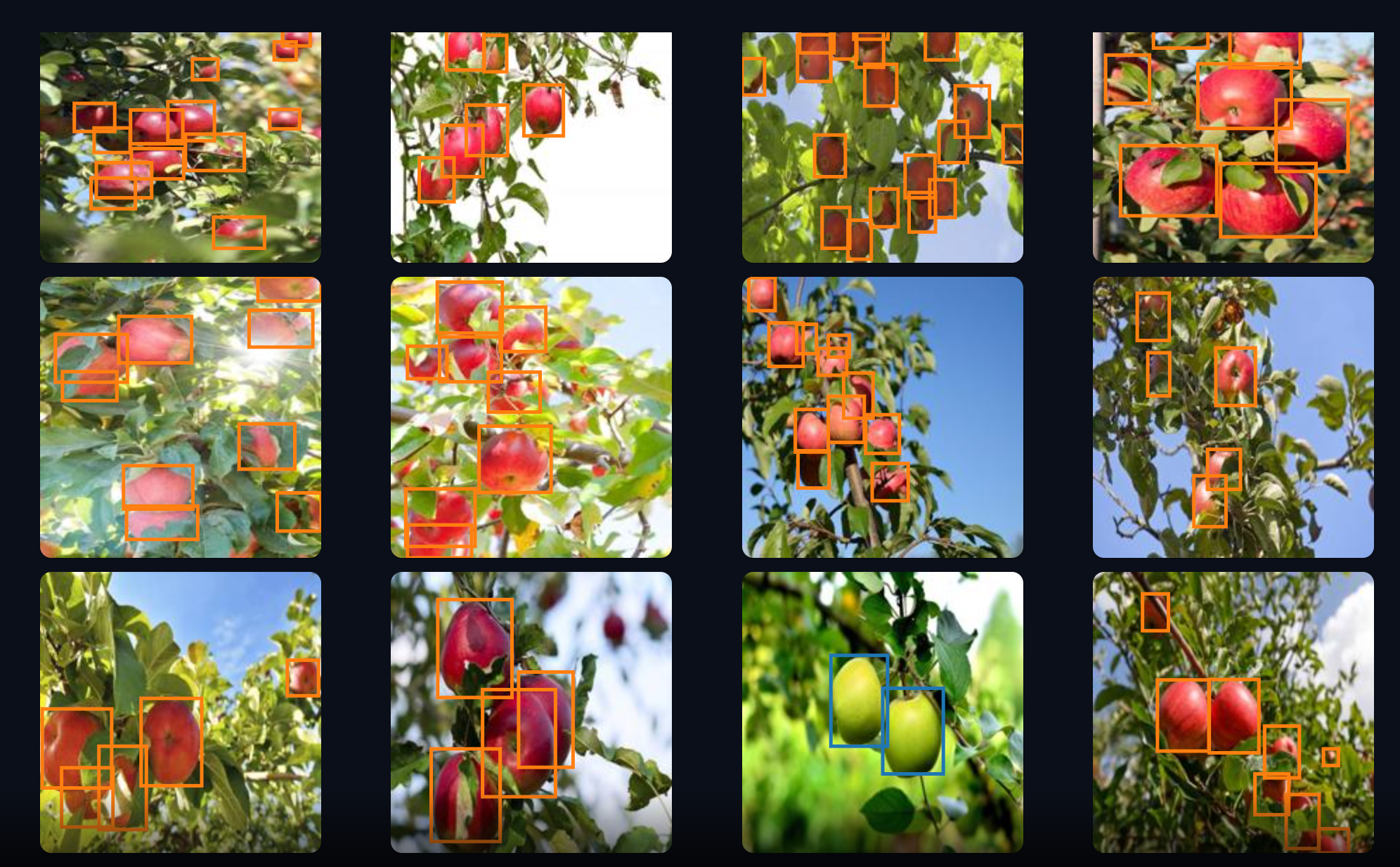
Top 4 Computer Vision Problems & Solutions in Agriculture — Part 1
This blog post was originally published at Tenyks’ website. It is reprinted here with the permission of Tenyks. In Part 1 of this series, we highlight the 4 main issues you are likely to encounter in object detection datasets in agriculture. We begin by summarizing the challenges of applying AI to crop monitoring and yield […]
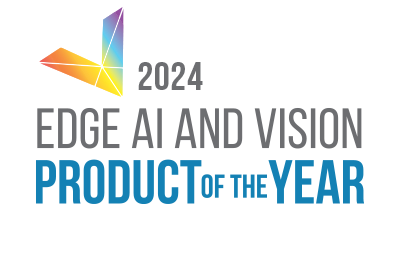
Edge AI and Vision Alliance™ Announces 2024 Edge AI and Vision Product of the Year™ and AI Innovation Award™ Winners
Awards Celebrate Innovation and Achievement in Computer Vision and Edge AI SANTA CLARA, CALIFORNIA, UNITED STATES OF AMERICA, May 23, 2024 /EINPresswire.com/ — The Edge AI and Vision Alliance today announced the 2024 winners of the Edge AI and Vision Product of the Year Awards and the AI Innovation Awards. The Edge AI and Vision […]
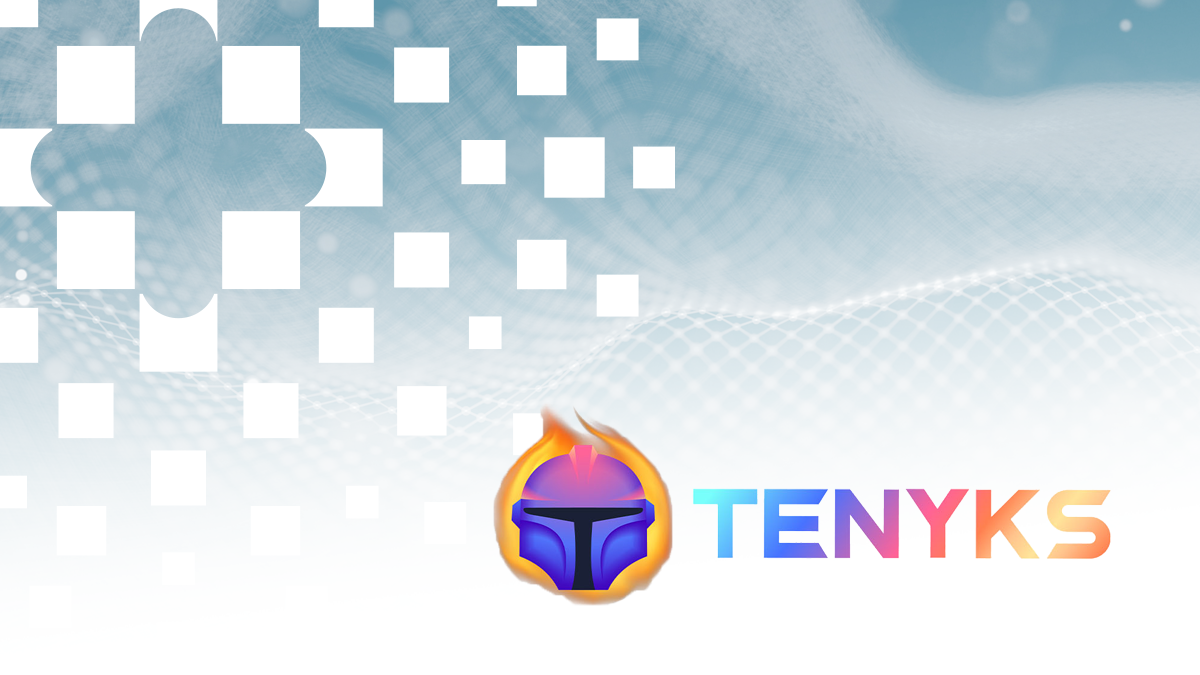
2024 Edge AI and Vision Product of the Year Award Winner Showcase: Tenyks (Edge AI Developer Tools)
Tenyks’ Data-Centric CoPilot for Vision is the 2024 Edge AI and Vision Product of the Year Award Winner in the Edge AI Developer Tools category. The Data-Centric CoPilot for Vision platform helps computer vision teams develop production-ready models 8x faster. The platform enables machine learning (ML) teams to mine edge cases, failure patterns and annotation […]
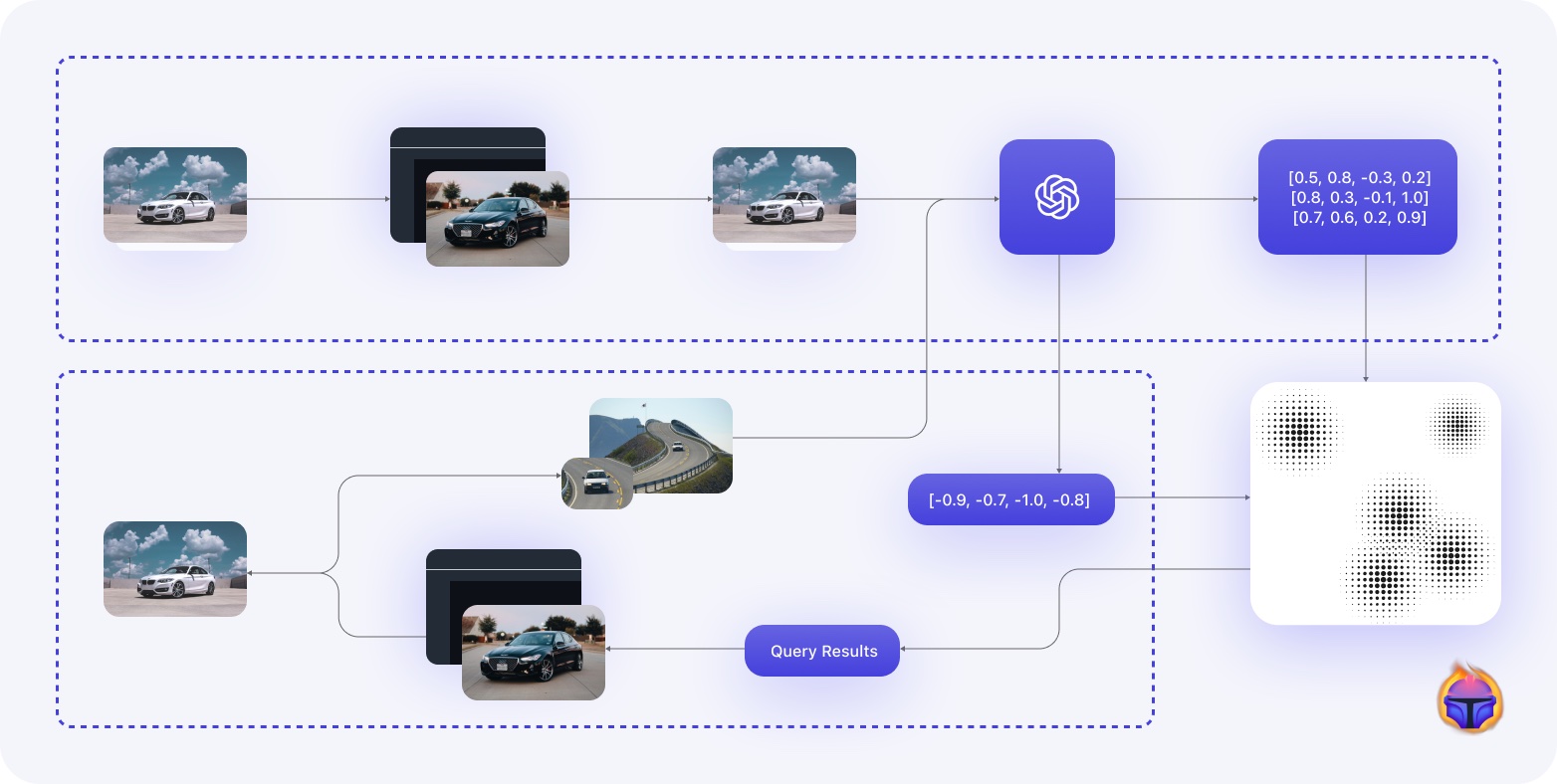
Unlocking the Potential of Unlabelled Data with Zero Shot Models and Vector Databases
This blog post was originally published at Tenyks’ website. It is reprinted here with the permission of Tenyks. Search and retrieval of thousands or even millions of images is a challenging task, especially when the data lacks explicit labels. Traditional search techniques rely on keywords, tags, or other annotations to match queries with relevant items. […]
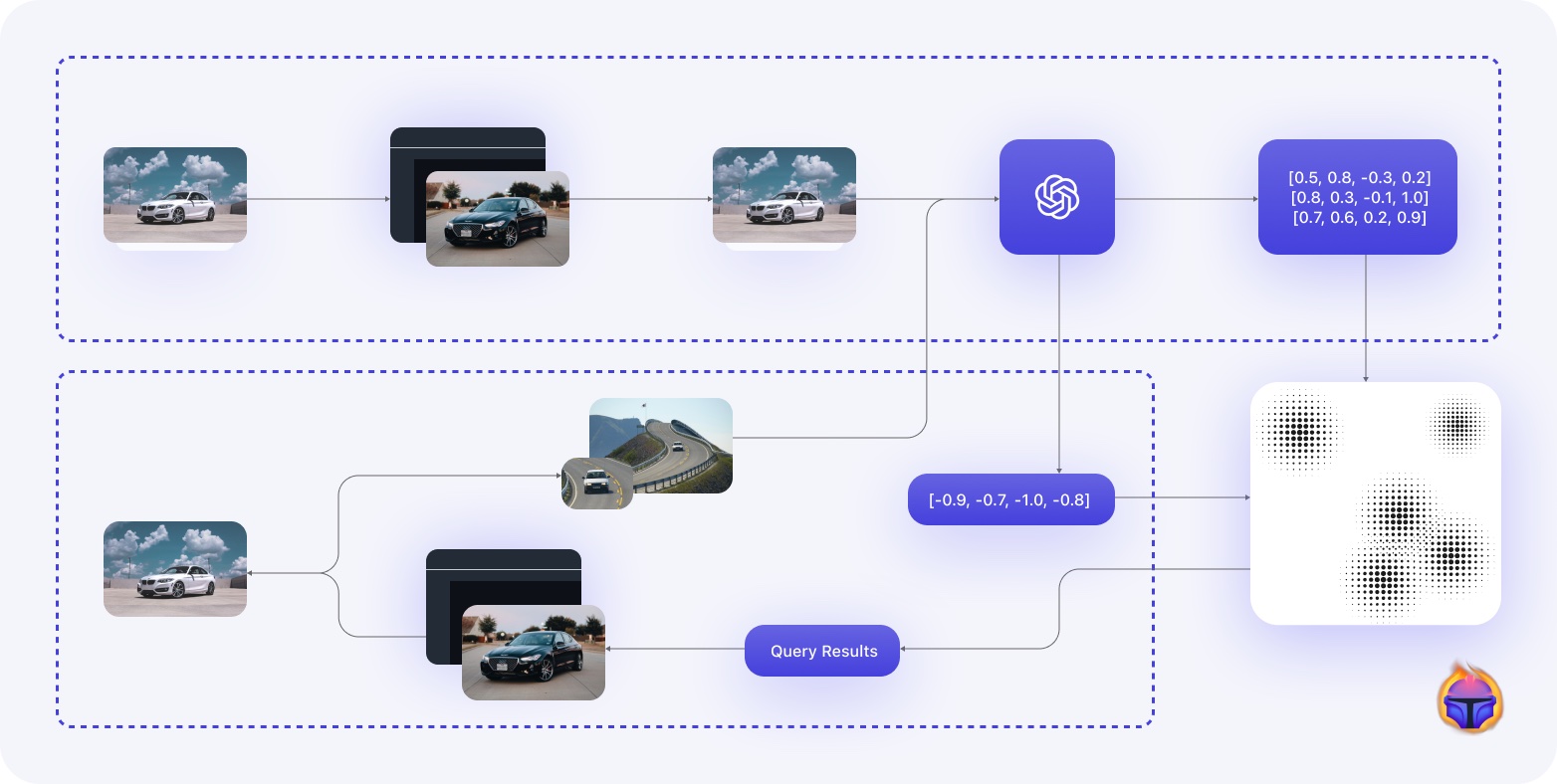
Multi-modal Image Search with Embeddings and Vector Databases
This blog post was originally published at Tenyks’ website. It is reprinted here with the permission of Tenyks. Use embeddings with vector databases to perform multi-modal search on images. Embeddings are a powerful way to represent and capture the semantic meaning and relationships between data points in a vector space. While word embeddings in NLP […]
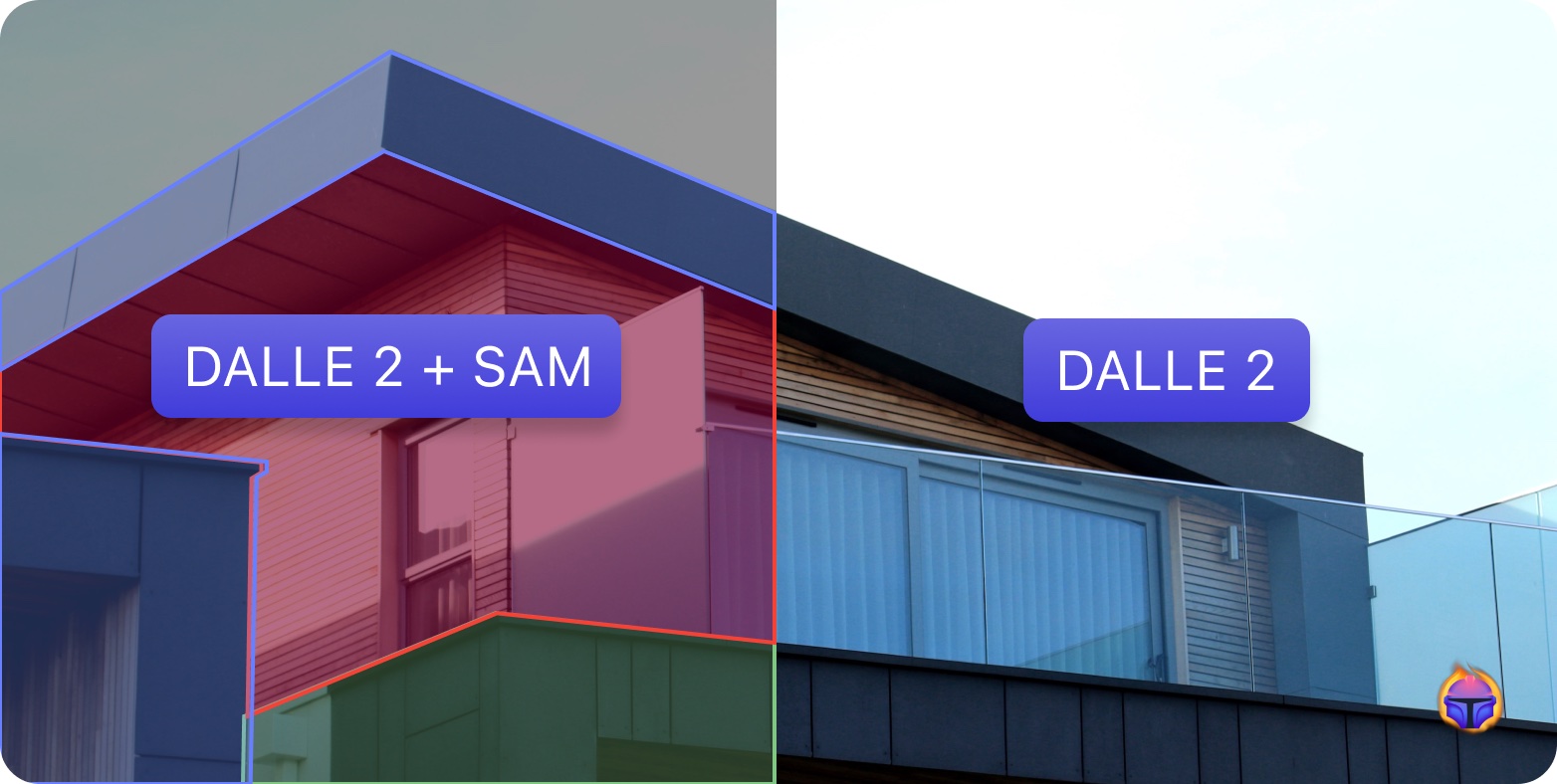
The Foundation Models Reshaping Computer Vision
This article was originally published at Tenyks’ website. It is reprinted here with the permission of Tenyks. Learn about the Foundation Models — for object classification, object detection, and segmentation — that are redefining Computer Vision. Foundation models have come to computer vision! Initially limited to language tasks, foundation models can now serve as the backbone of computer […]
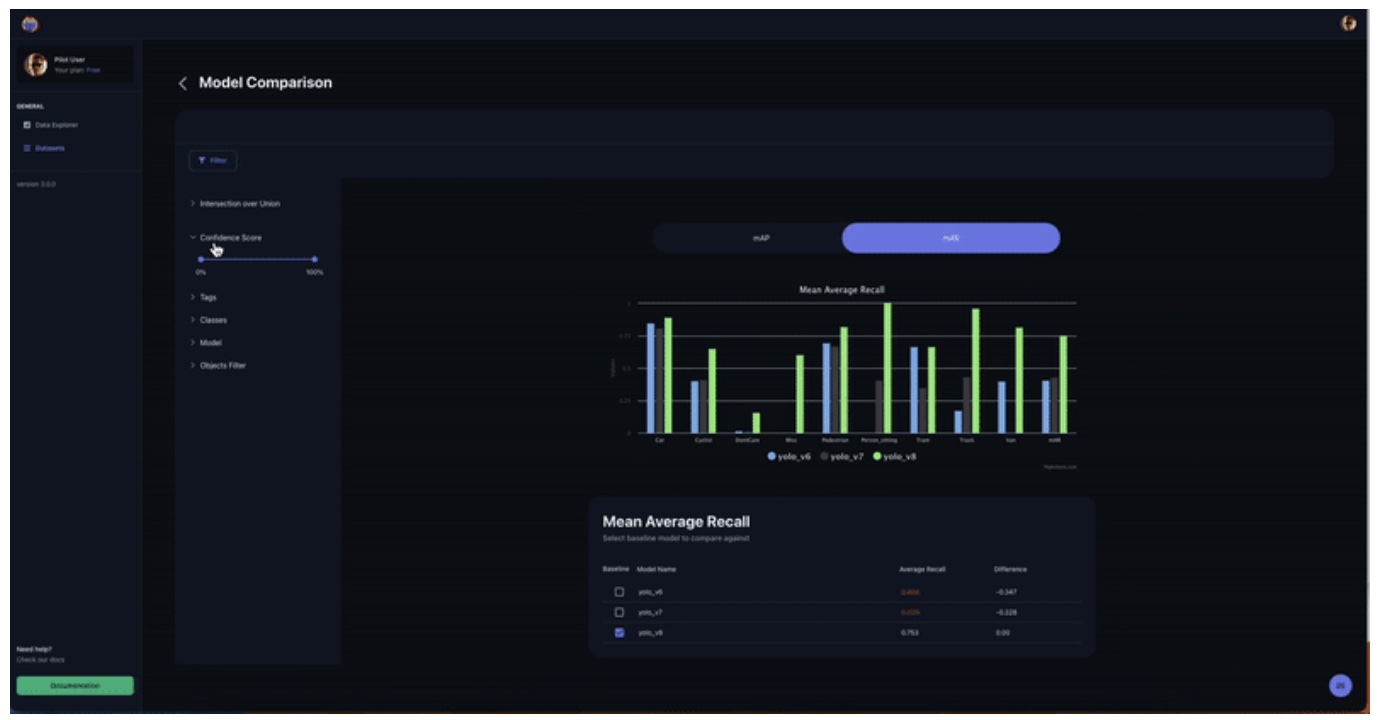
NVIDIA TAO Toolkit “Zero to Hero”: A Simple Guide for Model Comparison in Object Detection
This article was originally published at Tenyks’ website. It is reprinted here with the permission of Tenyks. In Part 2 of our NVIDIA TAO Toolkit series, we describe & address the common challenges of model deployment, in particular edge deployment. We explore practical solutions to these challenges, especially on the issues surrounding model comparison. Here […]
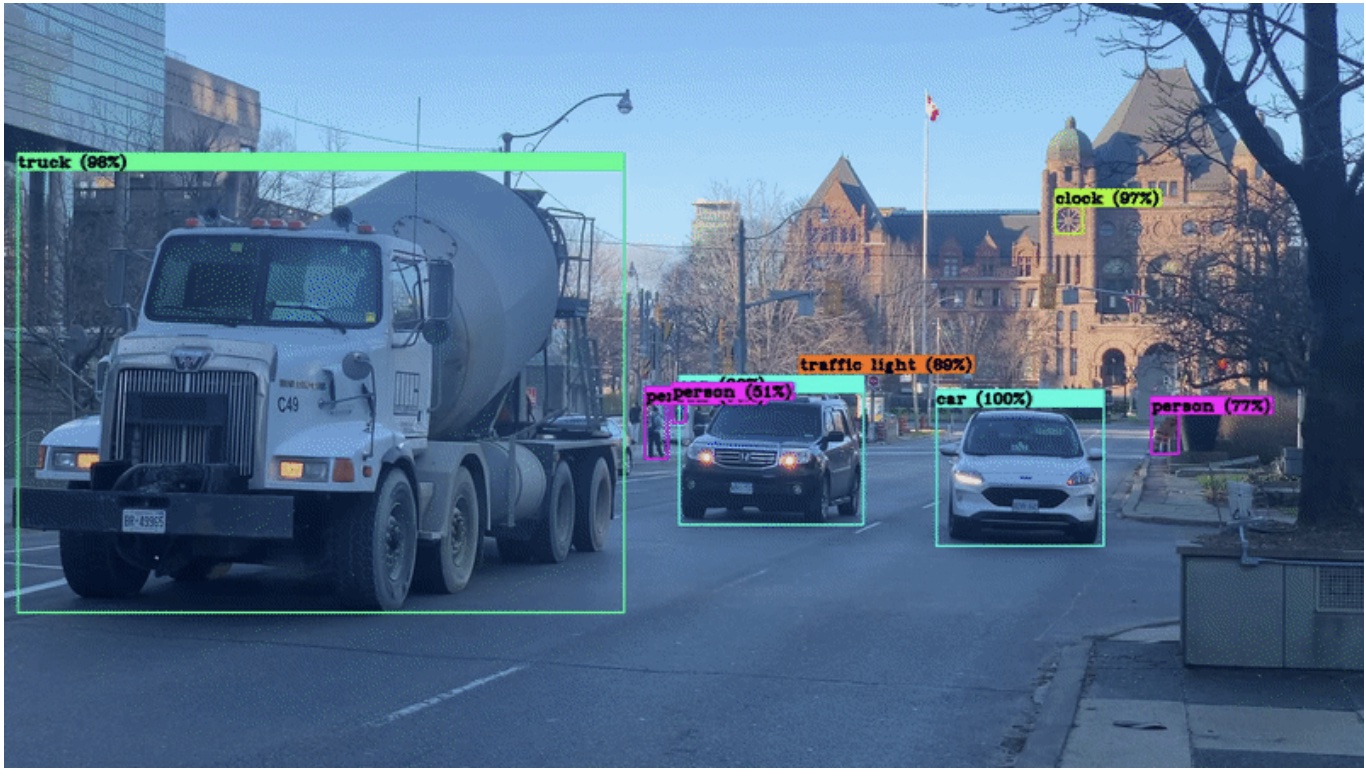
NVIDIA TAO Toolkit “Zero to Hero”: Setup Tips and Tricks
This article was originally published at Tenyks’ website. It is reprinted here with the permission of Tenyks. A quick setup guide for an NVIDIA TAO Toolkit (v3 & v4) object detection pipeline for edge computing, including tips & tricks and common pitfalls. This article will help you setup an NVIDIA TAO Toolkit (v3 & v4) […]
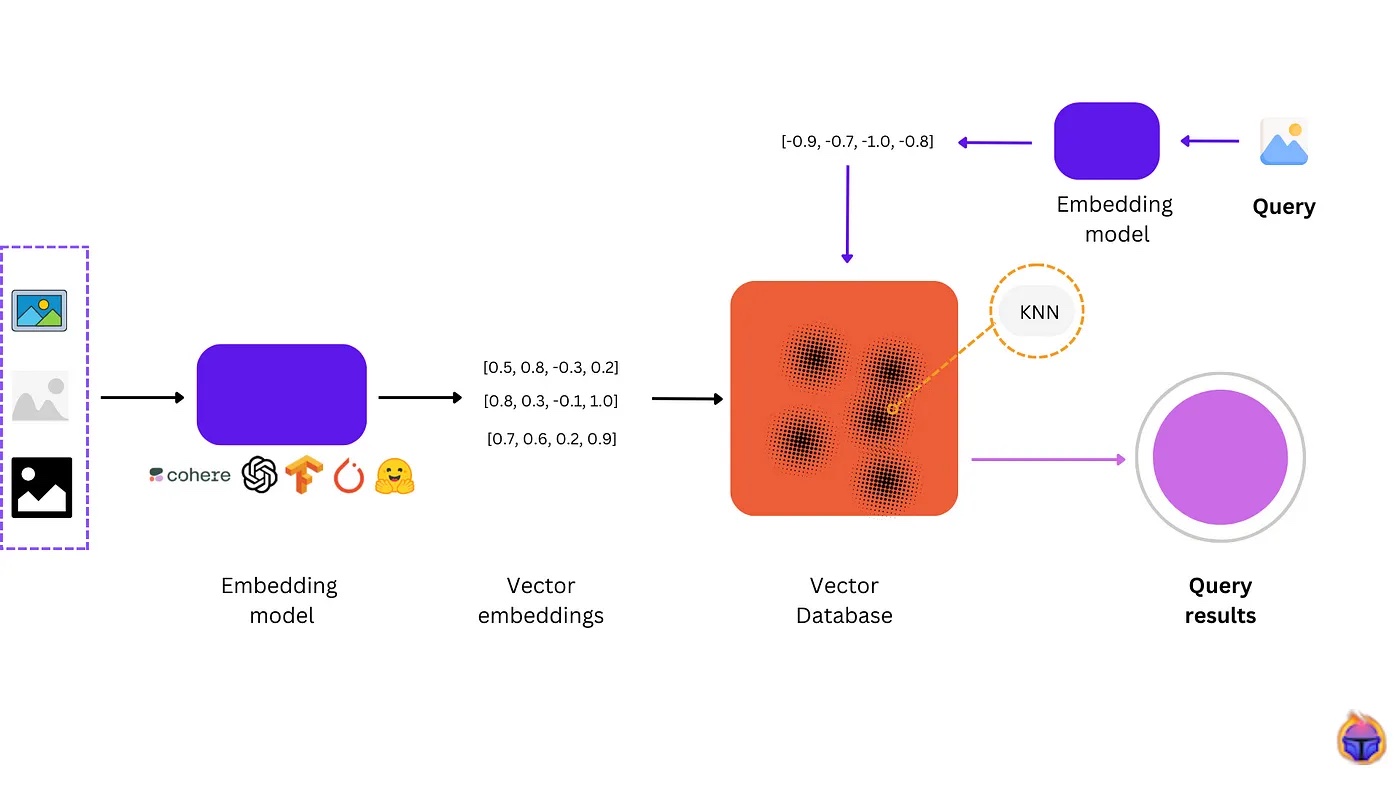
Vector Databases: Unlock the Potential of Your Data
This blog post was originally published at Tenyks’ website. It is reprinted here with the permission of Tenyks. In the field of artificial intelligence, vector databases are an emerging database technology that is transforming how we represent and analyze data by using vectors — multi-dimensional numerical arrays — to capture the semantic relationships between data […]
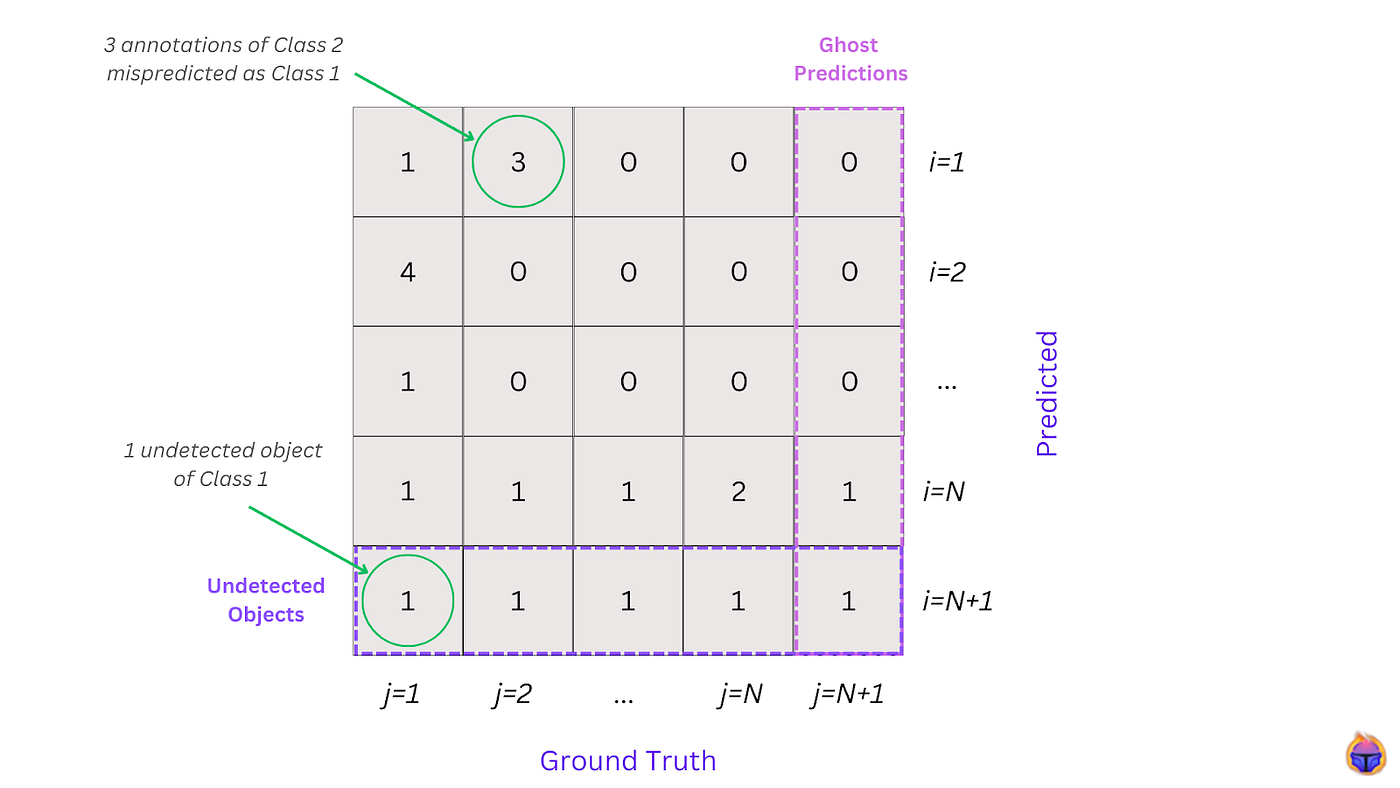
Multiclass Confusion Matrix for Object Detection
This blog post was originally published at Tenyks’ website. It is reprinted here with the permission of Tenyks. We introduce the Multiclass Confusion Matrix for Object Detection, a table that can help you perform failure analysis identifying otherwise unnoticeable errors, such as edge cases or non-representative issues in your data. In this article we introduce […]
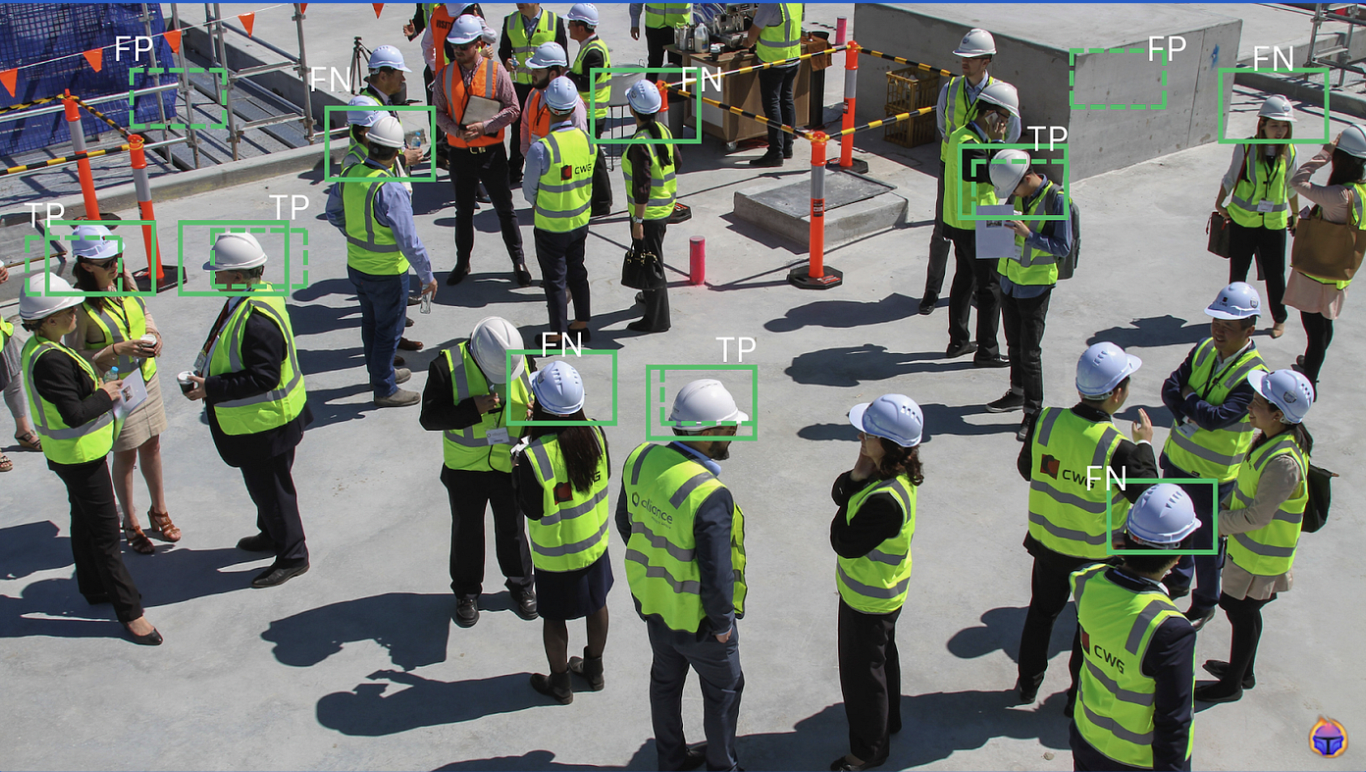
Mean Average Precision (mAP): Common Definition, Myths and Misconceptions
This blog post was originally published at Tenyks’ website. It is reprinted here with the permission of Tenyks. We break down and demystify common object detection metrics, including mean average precision (mAP) and mean average recall (mAR). This is Part 1 of our Tenyks Series on Object Detection Metrics. This post provides insights into how […]