M1 NPU Delivers Flexibility, Accuracy, Efficiency and Performance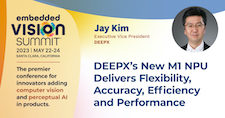
For a growing range of applications, deploying AI in the real world means running various models at the edge. Fortunately, powerful edge processors are increasingly capable of handling demanding AI workloads. But performance alone is not sufficient. To meet the needs of real-world applications, edge AI processors must also deliver flexibility, accuracy and efficiency. In this talk, Jay Kim, Executive Vice President of DEEPX, presents his company’s new M1 NPU. He explains how its unique architecture provides exceptional flexibility, enabling it to handle new DNN models and layer types with ease. Kim also shows how his company’s unique hardware/software co-design approach enables the M1 to deliver high performance with extreme cost- and energy-efficiency. And, finally, he shows how—despite its extreme efficiency—the new architecture achieves outstanding accuracy, comparable to that of GPUs.
Processing Raw Images Efficiently on the MAX78000 Neural Network Accelerator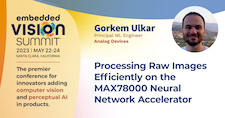
In this talk, Gorkem Ulkar, Principal ML Engineer at Analog Devices, presents alternative and more efficient methods of processing raw camera images using neural network accelerators. He begins by introducing Analog Devices’ convolutional neural network accelerator, MAX78000, and showing how it achieves superior performance and energy efficiency on a range of neural network inference tasks. In visual AI applications, cameras provide raw images not in the familiar RGB format, but in a Bayer format. In order to process these images using a neural network that was trained on RGB data, the camera images must be “de-Bayerized” to turn them into RGB images. The conventional way of performing this step is via interpolation. Unfortunately, this increases energy consumption and latency of the application since it cannot be performed by neural network accelerators. Ulkar presents alternative methods of performing this task using neural network accelerators and demonstrates the effectiveness of these techniques.
|
Sparking the Next Generation of Cloud-native Smart Camera Designs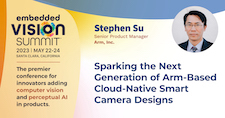
As enterprises and consumers increasingly adopt machine learning-enabled smart cameras, the expectations of these end users are becoming more sophisticated. In particular, smart camera users increasingly expect their deployed cameras to improve over time—for example, becoming more accurate and gaining new features. Traditionally, however, smart cameras that run machine learning at the edge have been difficult to upgrade. In this talk, Stephen Su, Senior Product Manager at Arm, explains how a cloud-native approach for running machine learning software at the edge enables smart camera developers to easily deploy improved models and new capabilities into existing, installed cameras. He uses application examples to illustrate the benefits of a cloud-native approach.
Develop Next-generation Camera Applications Using Snapdragon Computer Vision Technologies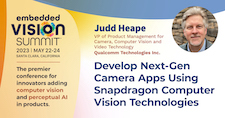
The Qualcomm Snapdragon mobile platform powers the world’s best smartphones, XR headsets, PCs, wearables, automobiles and IoT products. These devices leverage the latest computer vision technologies that power Snapdragon’s ISP, AR/VR perception pipeline and advanced video capture features. In this talk, Judd Heape, VP of Product Management for Camera, Computer Vision and Video Technology at Qualcomm Technologies, uses real-world examples—with a focus on AR/VR products—to explore how Snapdragon developers harness these computer vision technologies to enable advanced use cases with premium features, performance boosts and power savings. He also shows how developers use the Snapdragon computer vision SDKs and their camera-centric APIs to tap Snapdragon’s amazing hardware computer vision technologies to create next-generation immersive applications.
|
Cadence Strengthens the Tensilica Vision and AI Software Partner Ecosystem for Advanced Automotive, Mobile, Consumer and IoT Applications
BrainChip’s Latest White Paper Examines a New Approach to Optimizing Time-series Data
Qualcomm Acquires Autotalks, a Fabless Semiconductor Company Focused on V2X Communications
STMicroelectronics Introduces an Inertial Module with a Certified ASIL B Software Library for a Broad Range of Automotive Applications
STRADVISION Receives TISAX AL3, the European Automotive Industry’s Top Information Security Management Standard
More News
|
Deci Deep Learning Development Platform (Best Edge AI Developer Tool)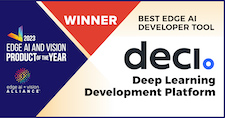
Deci’s Deep Learning Development Platform is the 2023 Edge AI and Vision Product of the Year Award winner in the Edge AI Developer Tools category. Deci’s deep learning development platform empowers AI developers with a new way to develop production grade computer vision models. With Deci, teams simplify and accelerate the development process with advanced tools to build, train, optimize, and deploy highly accurate and efficient models to any environment including edge devices. Models developed with Deci deliver a combination of high accuracy, speed and compute efficiency and therefore allow teams to unlock new applications on resource constrained edge devices and migrate workloads from cloud to edge. Also, Deci enables teams to shorten development time and lower operational costs by up to 80%. Deci’s platform is powered by its proprietary Automated Neural Architecture Construction (AutoNAC) technology, the most advanced algorithmic optimization engine that generates best-in-class deep learning model architectures for any vision-related task. With AutoNAC, teams can easily build custom, hardware-aware production grade models that deliver better than state-of-the-art performance.
Please see here for more information on Deci’s Deep Learning Development Platform. The Edge AI and Vision Product of the Year Awards celebrate the innovation of the industry’s leading companies that are developing and enabling the next generation of edge AI and computer vision products. Winning a Product of the Year award recognizes a company’s leadership in edge AI and computer vision as evaluated by independent industry experts. |