Deploy Your Embedded Vision Solution on Any Processor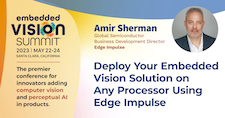
Vision-based product developers have a vast array of processors to choose from. Unfortunately, each processor has its own unique tool chain. This makes it difficult for developers to evaluate processor options and target different processors for different product needs. Fortunately, there’s Edge Impulse Studio—a universal development solution that is compatible with any vision-based processor. For example, when using Arm processors, developers can target Arm Cortex-M MCUs, the latest Cortex-M55, the Ethos U55 microNPU, and Cortex-A CPUs—along with the GPUs that are often used with them. Edge Impulse Studio also supports a wide range of specialized accelerators, such as the Renesas DRP-AI engine and neuromorphic processor cores. In this presentation, Amir Sherman, Global Semiconductor Business Development Director at Edge Impulse, shows the key features and benefits of Edge Impulse Studio and demonstrate how it enables developers to quickly evaluate processor platforms and easily deploy vision applications on a wide range of embedded hardware.
State-of-the-art Model Quantization and Optimization for Efficient Edge AI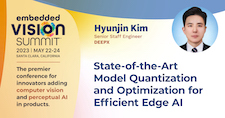
Extremely efficient edge AI requires more than efficient processors; it also requires tools capable of generating super-efficient software. In this talk, Hyunjin Kim, Senior Staff Engineer at DEEPX, explains and demonstrates how DEEPX’s DXNN SDK utilizes state-of-the-art optimization techniques to generate extremely efficient, accurate code for DEEPX’s new M1 neural processor. Kim begins by describing how the DXNN SDK uses hardware-aware, selective quantization to maintain high accuracy while achieving efficient DNN implementations. Next, he explains how the SDK maps DNN layer operations into processor micro-operations to provide both efficiency and flexibility. Kim also shows how the DEEPX SDK conserves memory by utilizing tiling, layer fusion and feature reuse. Finally, he illustrates the ease of use of the SDK by demonstrating the use of the DXNN SDK to implement a state-of-the-art model on the M1 NPU.
|
Image Sensors to Enable Low-cost and Low-power Computer Vision Applications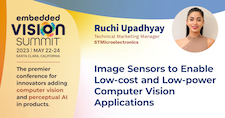
Advances in image sensor capabilities, such as improved imaging in low-light conditions, coupled with reduced footprint and lower power consumption, are enabling more and more systems to incorporate computer vision. In this presentation, Ruchi Upadhyay, Technical Marketing Manager at STMicroelectronics, illuminates the key image sensor specifications that computer vision system developers should focus on in order to deliver excellent end-user experiences and improve power efficiency. Upadhyay introduces key parameters such as quantum efficiency and context switching, explains their impact on system performance, and shows how to make the best use of innovative sensor capabilities to reduce the power consumption. She also touches on embedded image sensor features that can help system developers meet the challenging requirements of tomorrow’s devices.
Selecting Image Sensors for Embedded Vision Applications: Three Case Studies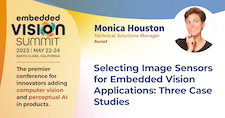
Selecting the appropriate type of image sensor is essential for reliable and accurate performance of vision applications. In this talk, Monica Houston, Technical Solutions Manager at Avnet, explores some of the critical factors to consider in selecting an image sensor, including shutter type, dynamic range, resolution and chromaticity. She explores the impact of these factors through three distinct use cases: defect detection, license plate recognition and crowd counting. For defect detection, Houston examines the advantages and disadvantages of monochrome image sensors. In license plate recognition, she highlights the importance of global shutter, pixel size, dynamic range and color space, providing a comprehensive introduction to key factors that contribute to successful recognition in varying light conditions. Lastly, she explores the trade-offs of high-resolution cameras in crowd counting applications and offers practical insights on developing fast and accurate machine learning models with high-resolution input.
|
Piera Systems Canāree Air Quality Monitor (Best Enterprise Edge AI End Product)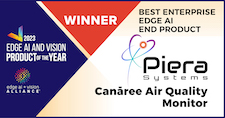
Piera Systems’ Canāree Air Quality Monitor is the 2023 Edge AI and Vision Product of the Year Award winner in the Enterprise Edge AI End Products category. The Canāree family of air quality monitors (AQMs) are compact, highly accurate, and easy to use. The Canāree AQMs are also the most innovative, cost-effective air quality monitors owing largely to the quality of the data they produce which in turn helps classify, and in some cases identify, specific pollutants. Identifying when someone is vaping in a school bathroom or a hotel room is a good example of this technology in action. Classification of pollutants is done by employing AI/ML techniques on the highly accurate data produced by the Canāree AQMs. This is the only low-cost AQM in the world with such a capability. The Canāree AQMs measure various environmental factors including particles, temperature, pressure, humidity, and VOCs. While many similar products exist in the market, Canāree is the only one with a highly accurate particle sensor which uniquely sets it apart. Canāree AQMs measure particles ranging from 10 microns in size all the way down to 100 nanometers, a unique capability in this industry. This particle data is distributed into seven size “bins” and these data bins are the foundation for its classification capabilities.
Please see here for more information on Piera Systems’ Canāree Air Quality Monitor. The Edge AI and Vision Product of the Year Awards celebrate the innovation of the industry’s leading companies that are developing and enabling the next generation of edge AI and computer vision products. Winning a Product of the Year award recognizes a company’s leadership in edge AI and computer vision as evaluated by independent industry experts. |