Dear Colleague,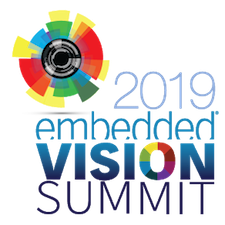
The 2019 Embedded Vision Summit, held last month in Santa Clara, California, brought together over 1,200 attendees to learn about technologies, challenges, techniques and opportunities for products using computer vision and visual AI. Summit presentation slides are now available for download in PDF format as a single ZIP file. Also now available are the videos of the keynote presentation from Google's Pete Warden along with the Day 1 and Day 2 introductory remarks from Embedded Vision Alliance founder Jeff Bier. Additional recordings of the event's various presentations and demonstrations will appear on the website in the coming weeks, with availability announced in the Alliance's newsletters.
Congratulations to the Alliance's 2019 Vision Product of the Year Award winners: Horizon Robotics' Horizon Matrix (Best Automotive Solution), Infineon Technologies' IRS2381C 3D Image Sensor (Best Sensor), Intel's OpenVINO Toolkit (Best Developer Tools), MediaTek's Helio P90 (Best AI Technology), Morpho's Video Processing Software (Best Software or Algorithm), Synopsys's EV6x Embedded Vision Processors with Safety Enhancement Package (Best Processor), and Xilinx's AI Platform (Best Cloud Solution). Congratulations as well to the Alliance's 2019 Vision Tank Start-up Competition winners: Strayos (Judges' Choice) and BlinkAI Technologies (Audience Choice).
Also check out the new product announcements from multiple Alliance Member companies at the Summit:
Mark your calendars and plan to attend next year's Summit, May 18-21, 2020, once again at the Santa Clara Convention Center. I'll see you there!
Brian Dipert
Editor-In-Chief, Embedded Vision Alliance
|
From Feature Engineering to Network Engineering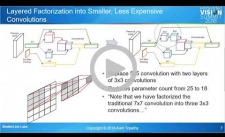
The availability of large labeled image datasets is tilting the balance in favor of “network engineering” instead of “feature engineering”. Hand-designed features dominated recognition tasks in the past, but now features can be automatically learned by back-propagating errors through the layers of a hierarchical “network” of feature maps. As a result, we’re seeing a plethora of network topologies that satisfy design objectives such as reduced parameter count, lower compute complexity, and faster learning. Certain core network building-blocks have emerged, such as split-transform-merge (as in the Inception module), skipping layers (as in Resnet, Densenet and its variants), weight-sharing across two independent networks for similarity learning (as in a Siamese network), and encoder/decoder network topologies for segmentation (as in Unet/Linknet). In this presentation, Auro Tripathy, Founding Principal at ShatterLine Labs, highlights these topologies from a feature representation and a feature learning angle, and shows how they are succinctly implemented in the Keras high-level deep learning framework (with brief Python code snippets).
Utilizing Neural Networks to Validate Display Content in Mission Critical Systems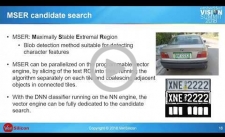
Mission critical display systems in aerospace, automotive and industrial markets require validation of the content presented to the user, in order to enable detection of potential failures and triggering of failsafe mechanisms. Traditional validation methods are based on pixel-perfect matching between expected and presented content. As user interface (UI) designs in these systems become more elaborate, the traditional validation methods become obsolete, and must be replaced with more robust methods that can recognize the mission critical information in a dynamic UI. In this talk, Shang-Hung Lin, Vice President of Vision and Imaging Products at VeriSilicon, explores limitations of the current content integrity checking systems and how they can be overcome by deployment of neural network pattern classification in the display pipeline. He also discusses the downscaling of these neural networks to run efficiently in a functionally safe microcontroller environment, and the requirements imposed on such solutions by the safety standards enforced in these domains.
|
Energy-efficient Processors Enable the Era of Intelligent Devices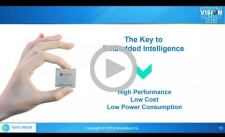
Artificial intelligence is making waves and headlines. New algorithms, applications and companies are emerging fast. Deep-learning-based systems, trained with massive amounts of data using supercomputers, are more capable than ever before. The most important opportunity for AI is supercharging the Internet of Things, making the “things” themselves smarter. With AI, edge devices gain the ability to sense, interpret and react intelligently to the world around them – creating the Intelligent Internet of Things (IIoT). To achieve this goal, it is essential that we make AI much more efficient, so that small, inexpensive, low-power systems can incorporate sophisticated AI to improve people’s lives. In this talk, Dr. Ren Wu, Founder and CEO of NovuMind, shares his perspective on the opportunity for AI at the edge, and explains how NovuMind is tackling this opportunity using domain-specific processor architectures, designed from the ground up for efficient AI, coupled with algorithms tailored for these processors.
The Journey and Sunrise Processors: Leading-Edge Performance for Embedded AI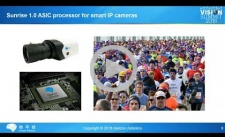
As the nature of computation changes from logic to artificial intelligence, there’s a revolution happening at the edge. According to Kai Yu, Founder and CEO of Horizon Robotics, a new type of processor, is required for this post-Moore’s-law era. Horizon Robotics, a leading technology powerhouse in embedded AI, is dedicated to providing embedded AI solutions including algorithms, chips and cloud. In this presentation, Dr. Yu presents Horizon’s embedded AI computer vision processors, Journey and Sunrise. Based on Horizon Robotics’ Brain Processing Unit (BPU), these processors power smart cars and smart cameras.
|