LLMs and MLLMs
The past decade-plus has seen incredible progress in practical computer vision. Thanks to deep learning, computer vision is dramatically more robust and accessible, and has enabled compelling capabilities in thousands of applications, from automotive safety to healthcare. But today’s widely used deep learning techniques suffer from serious limitations. Often, they struggle when confronted with ambiguity (e.g., are those people fighting or dancing?) or with challenging imaging conditions (e.g., is that shadow in the fog a person or a shrub?). And, for many product developers, computer vision remains out of reach due to the cost and complexity of obtaining the necessary training data, or due to lack of necessary technical skills.
Recent advances in large language models (LLMs) and their variants such as vision language models (VLMs, which comprehend both images and text), hold the key to overcoming these challenges. VLMs are an example of multimodal large language models (MLLMs), which integrate multiple data modalities such as language, images, audio, and video to enable complex cross-modal understanding and generation tasks. MLLMs represent a significant evolution in AI by combining the capabilities of LLMs with multimodal processing to handle diverse inputs and outputs.
The purpose of this portal is to facilitate awareness of, and education regarding, the challenges and opportunities in using LLMs, VLMs, and other types of MLLMs in practical applications — especially applications involving edge AI and machine perception. The content that follows (which is updated regularly) discusses these topics. As a starting point, we encourage you to watch the recording of the symposium “Your Next Computer Vision Model Might be an LLM: Generative AI and the Move From Large Language Models to Vision Language Models“, sponsored by the Edge AI and Vision Alliance. A preview video of the symposium introduction by Jeff Bier, Founder of the Alliance, follows:
If there are topics related to LLMs, VLMs or other types of MLLMs that you’d like to learn about and don’t find covered below, please email us at [email protected] and we’ll consider adding content on these topics in the future.
View all LLM and MLLM Content
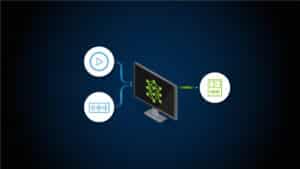
An Easy Introduction to Multimodal Retrieval-augmented Generation for Video and Audio
This blog post was originally published at NVIDIA’s website. It is reprinted here with the permission of NVIDIA. Building a multimodal retrieval augmented generation (RAG) system is challenging. The difficulty comes from capturing and indexing information from across multiple modalities, including text, images, tables, audio, video, and more. In our previous post, An Easy Introduction
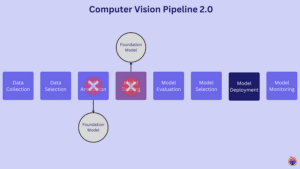
Computer Vision Pipeline v2.0
This blog post was originally published at Tenyks’ website. It is reprinted here with the permission of Tenyks. In the realm of computer vision, a shift is underway. This article explores the transformative power of foundation models, digging into their role in reshaping the entire computer vision pipeline. It also demystifies the hype behind the
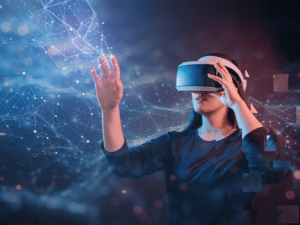
Virtual and Augmented Reality: The Rise and Drawbacks of AR
While being closed off from the real world is an experience achievable with virtual reality (VR) headsets, augmented reality (AR) offers images and data combined with real-time views to create an enriched and computing-enhanced experience. IDTechEx‘s portfolio of reports, including “Optics for Virtual, Augmented and Mixed Reality 2024-2034: Technologies, Players and Markets“, explore the latest
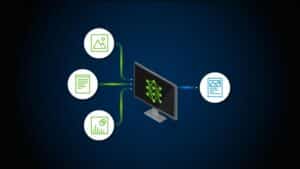
An Easy Introduction to Multimodal Retrieval-augmented Generation
This blog post was originally published at NVIDIA’s website. It is reprinted here with the permission of NVIDIA. A retrieval-augmented generation (RAG) application has exponentially higher utility if it can work with a wide variety of data types—tables, graphs, charts, and diagrams—and not just text. This requires a framework that can understand and generate responses
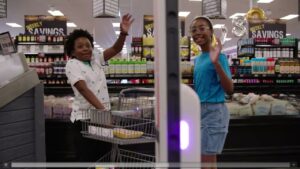
“Using Computer Vision-powered Robots to Improve Retail Operations,” a Presentation from Simbe Robotics
Durgesh Tiwari, VP of Hardware Systems, R&D at Simbe Robotics, presents the “Using Computer Vision-powered Robots to Improve Retail Operations” tutorial at the December 2024 Edge AI and Vision Innovation Forum. In this presentation, you’ll learn how Simbe Robotics’ AI- and CV-enabled robot, Tally, provides store operators with real-time intelligence to improve inventory management, streamline
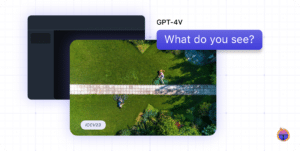
Amid the Rise of LLMs, is Computer Vision Dead?
This blog post was originally published at Tenyks’ website. It is reprinted here with the permission of Tenyks. The field of computer vision has seen incredible progress, but some believe there are signs it is stalling. At the International Conference on Computer Vision 2023 workshop “Quo Vadis, Computer Vision?”, researchers discussed what’s next for computer
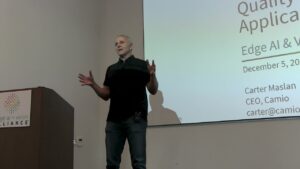
“Vision Language Models for Regulatory Compliance, Quality Control and Safety Applications,” a Presentation from Camio
Carter Maslan, CEO of Camio, presents the “Vision Language Models for Regulatory Compliance, Quality Control and Safety Applications” tutorial at the December 2024 Edge AI and Vision Innovation Forum. In this presentation, you’ll learn how vision language models interpret policy text to enable much more sophisticated understanding of scenes and human behavior compared with current-generation
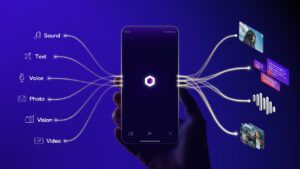
Snapdragon Summit’s AI Highlights: A Look at the Future of On-device AI
This blog post was originally published at Qualcomm’s website. It is reprinted here with the permission of Qualcomm. Qualcomm Technologies sets new standards in AI performance for its latest mobile, automotive and Qualcomm AI Hub advancements Our annual Snapdragon Summit wrapped up with exciting new announcements centered on the future of on-device artificial intelligence (AI).
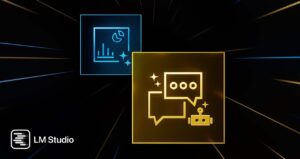
How to Accelerate Larger LLMs Locally on RTX With LM Studio
This blog post was originally published at NVIDIA’s website. It is reprinted here with the permission of NVIDIA. GPU offloading makes massive models accessible on local RTX AI PCs and workstations. Editor’s note: This post is part of the AI Decoded series, which demystifies AI by making the technology more accessible, and showcases new hardware,
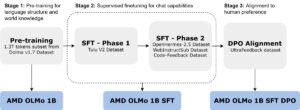
Introducing the First AMD 1B Language Models: AMD OLMo
This blog post was originally published at AMD’s website. It is reprinted here with the permission of AMD. In recent years, the rapid development of artificial intelligence technology, especially the progress in large language models (LLMs), has garnered significant attention and discussion. From the emergence of ChatGPT to subsequent models like GPT-4 and Llama, these
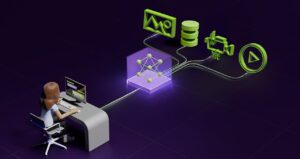
Give AI a Look: Any Industry Can Now Search and Summarize Vast Volumes of Visual Data
This blog post was originally published at NVIDIA’s website. It is reprinted here with the permission of NVIDIA. Accenture, Dell Technologies and Lenovo are among the companies tapping a new NVIDIA AI Blueprint to develop visual AI agents that can boost productivity, optimize processes and create safer spaces. Enterprises and public sector organizations around the
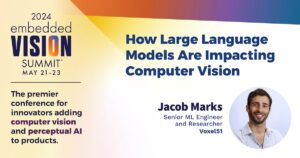
“How Large Language Models Are Impacting Computer Vision,” a Presentation from Voxel51
Jacob Marks, Senior ML Engineer and Researcher at Voxel51, presents the “How Large Language Models Are Impacting Computer Vision” tutorial at the May 2024 Embedded Vision Summit. Large language models (LLMs) are revolutionizing the way we interact with computers and the world around us. However, in order to truly understand… “How Large Language Models Are
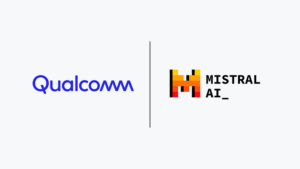
Qualcomm and Mistral AI Partner to Bring New Generative AI Models to Edge Devices
Highlights: Qualcomm announces collaboration with Mistral AI to bring Mistral AI’s models to devices powered by Snapdragon and Qualcomm platforms. Mistral AI’s new state-of-the-art models, Ministral 3B and Ministral 8B, are being optimized to run on devices powered by the new Snapdragon 8 Elite Mobile Platform, Snapdragon Cockpit Elite and Snapdragon Ride Elite, and Snapdragon
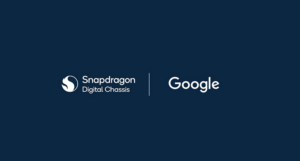
Qualcomm Announces Multi-year Strategic Collaboration with Google to Deliver Generative AI Digital Cockpit Solutions
Highlights: Qualcomm and Google will leverage Snapdragon Digital Chassis and Google’s in-vehicle technologies to produce a standardized reference framework for development of generative AI-enabled digital cockpits and software-defined vehicles (SDV). Qualcomm to lead go-to-market efforts for scaling and customization of joint solution with the broader automotive ecosystem. Companies’ collaboration demonstrates power of co-innovation, empowering automakers
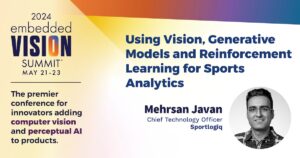
“Using Vision Systems, Generative Models and Reinforcement Learning for Sports Analytics,” a Presentation from Sportlogiq
Mehrsan Javan, Chief Technology Officer at Sportlogiq, presents the “Using Vision Systems, Generative Models and Reinforcement Learning for Sports Analytics” tutorial at the May 2024 Embedded Vision Summit. At a high level, sport analytics systems can be broken into two components: sensory data collection and analytical models that turn sensory… “Using Vision Systems, Generative Models
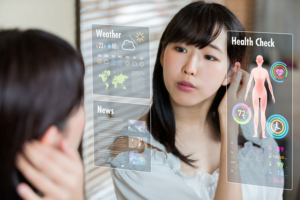
Exploring the Next Frontier of AI: Multimodal Systems and Real-time Interaction
This blog post was originally published at Qualcomm’s website. It is reprinted here with the permission of Qualcomm. Discover the state of the art in large multimodal models with Qualcomm AI Research In the realm of artificial intelligence (AI), the integration of senses — seeing, hearing and interacting — represents a frontier that is rapidly